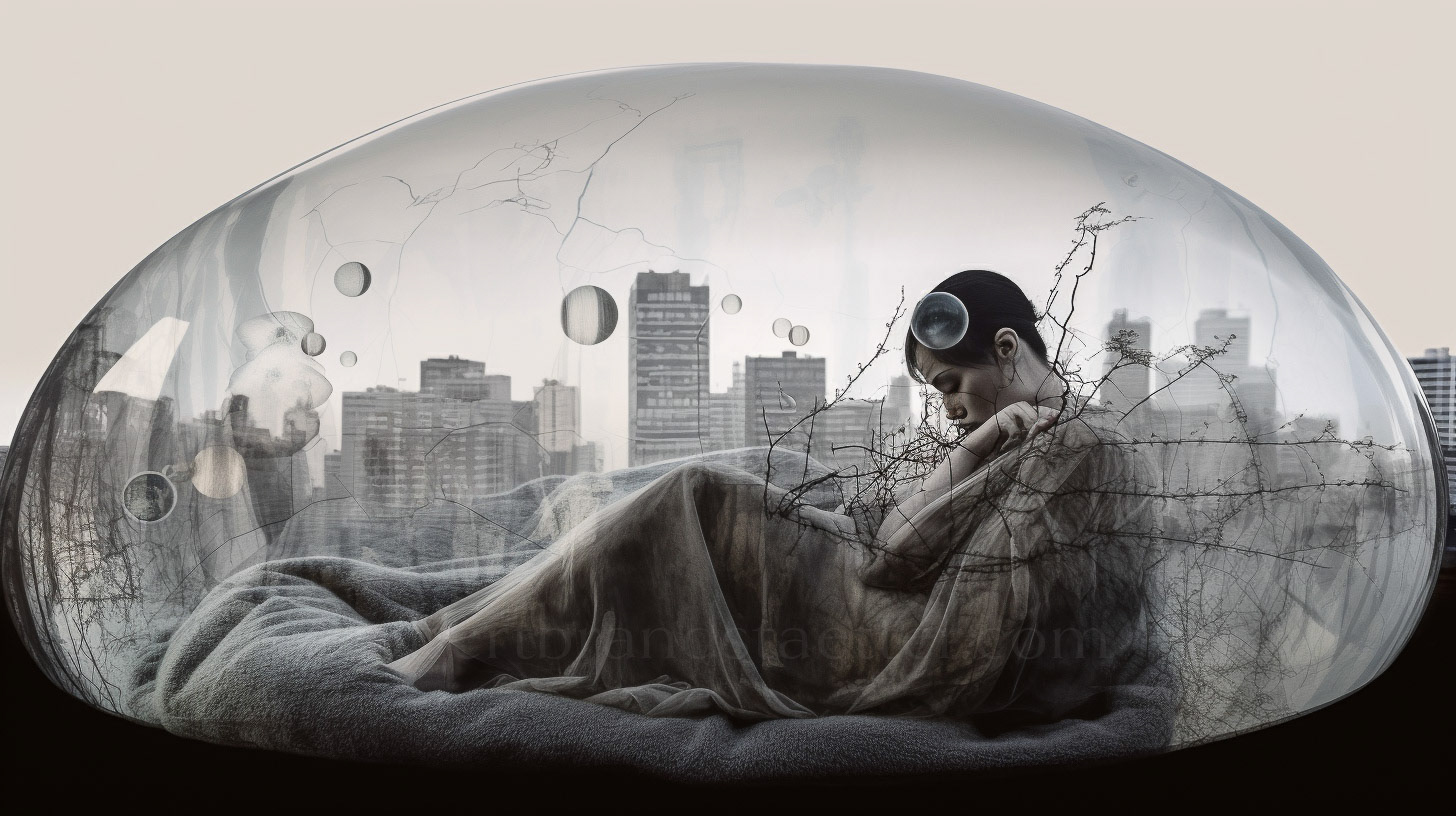
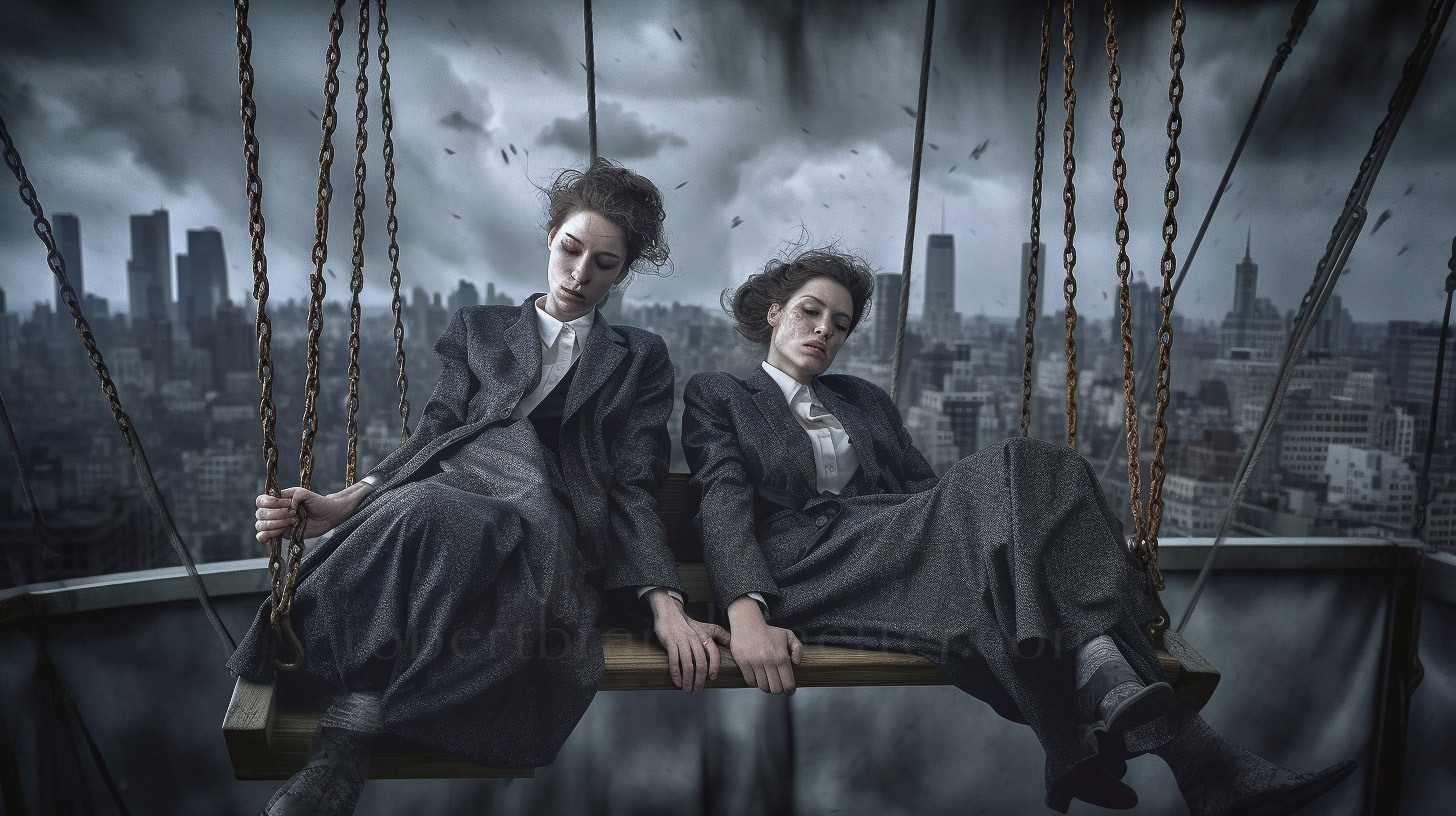
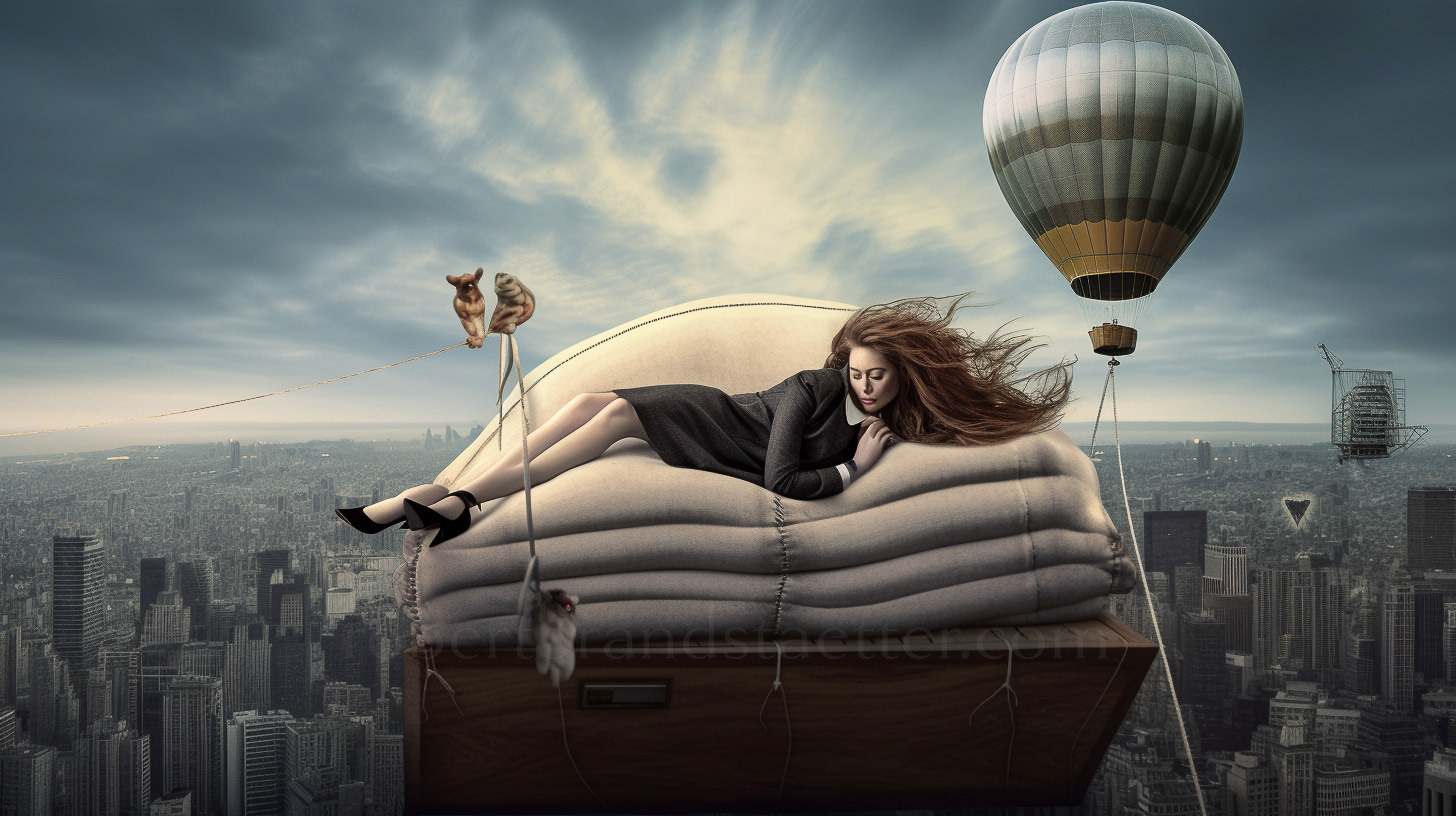
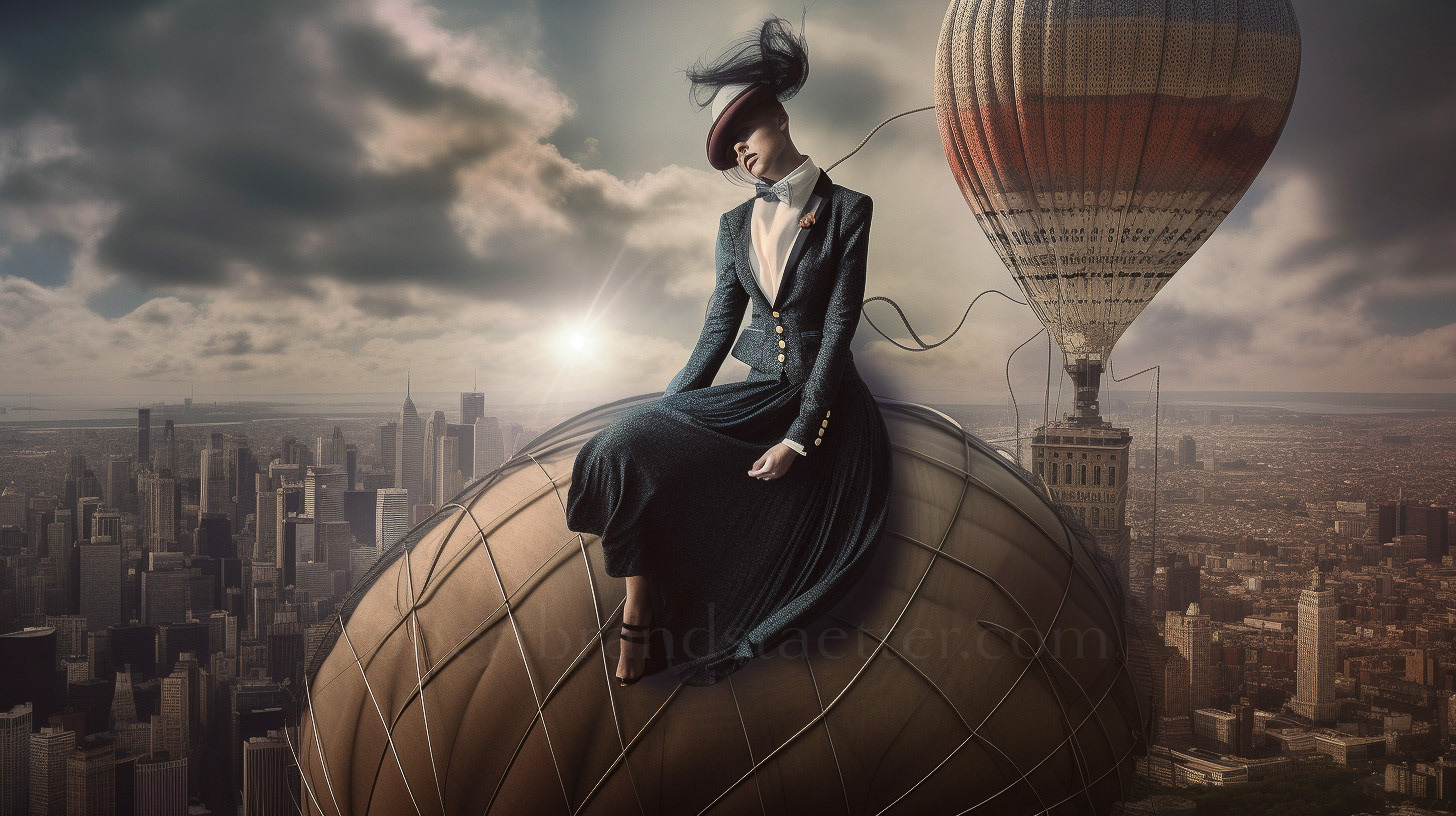
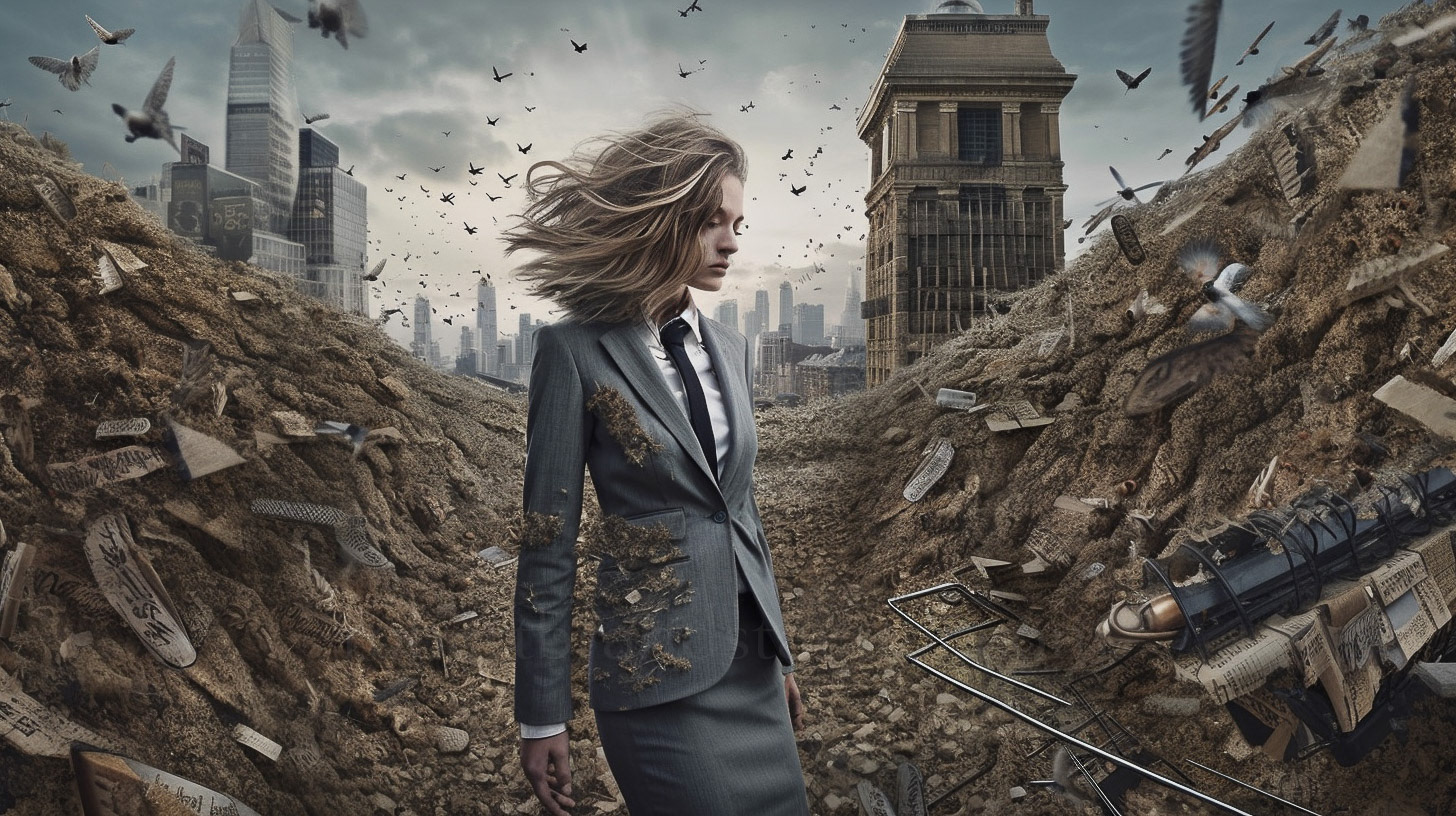
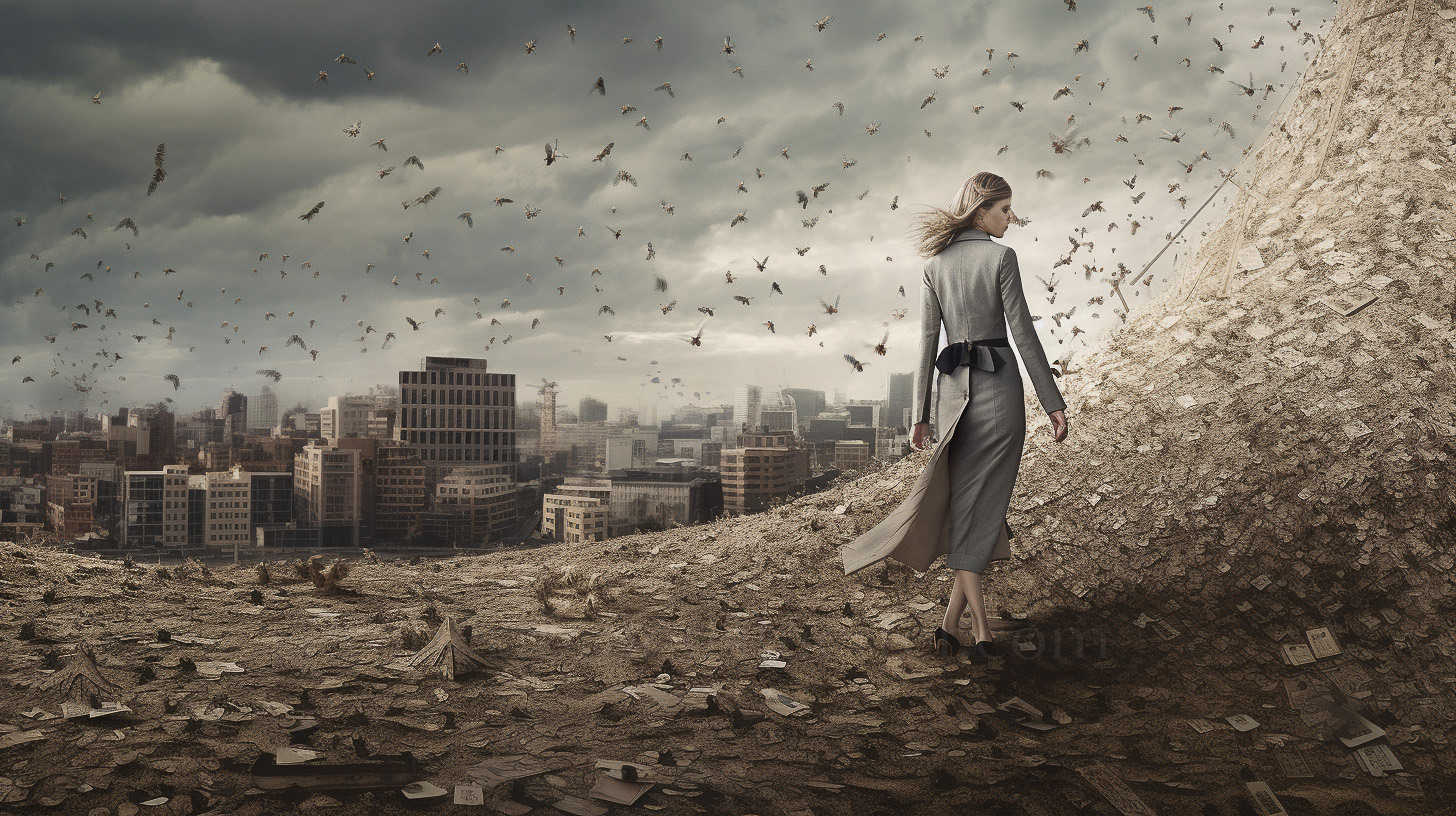
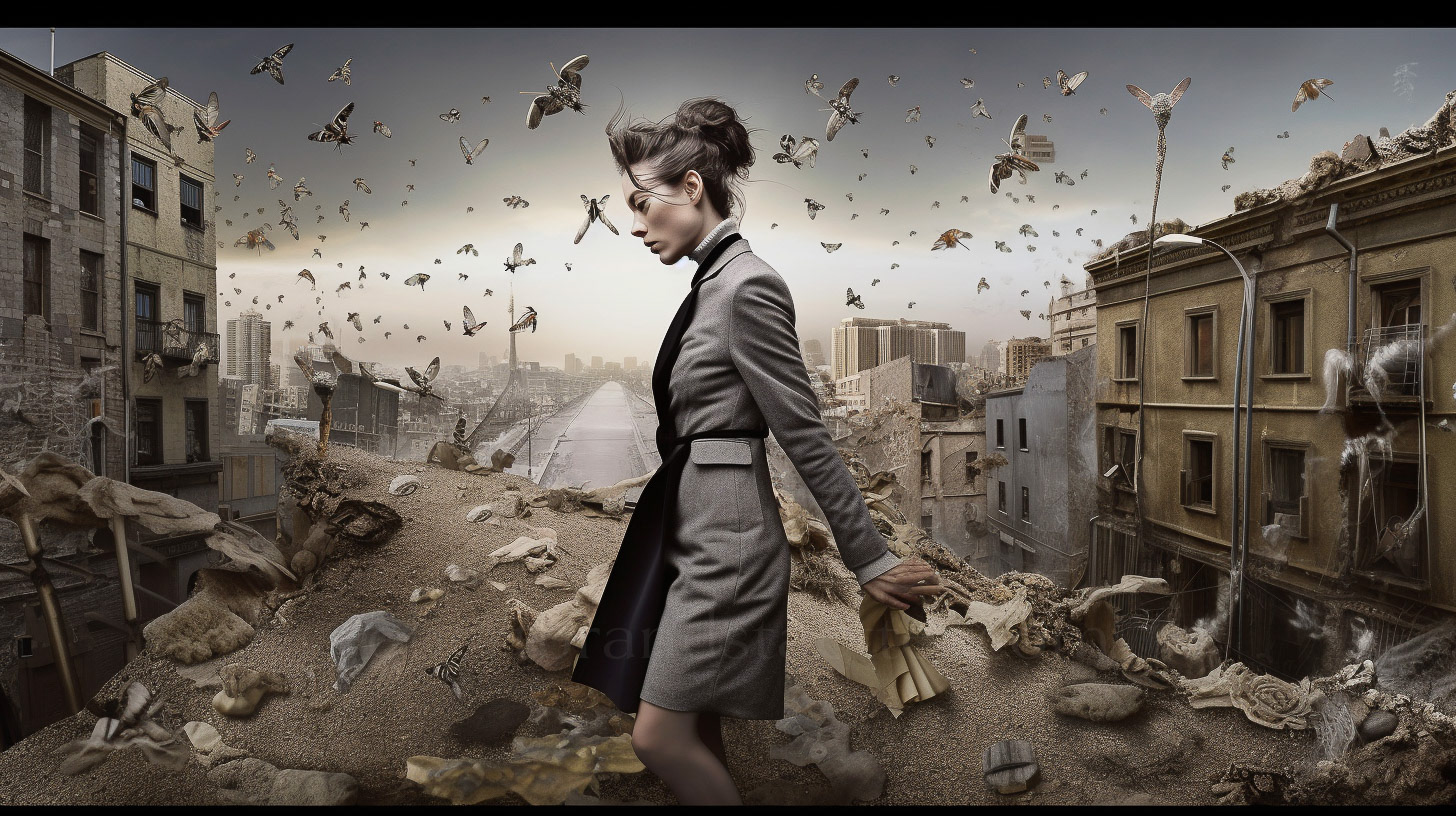
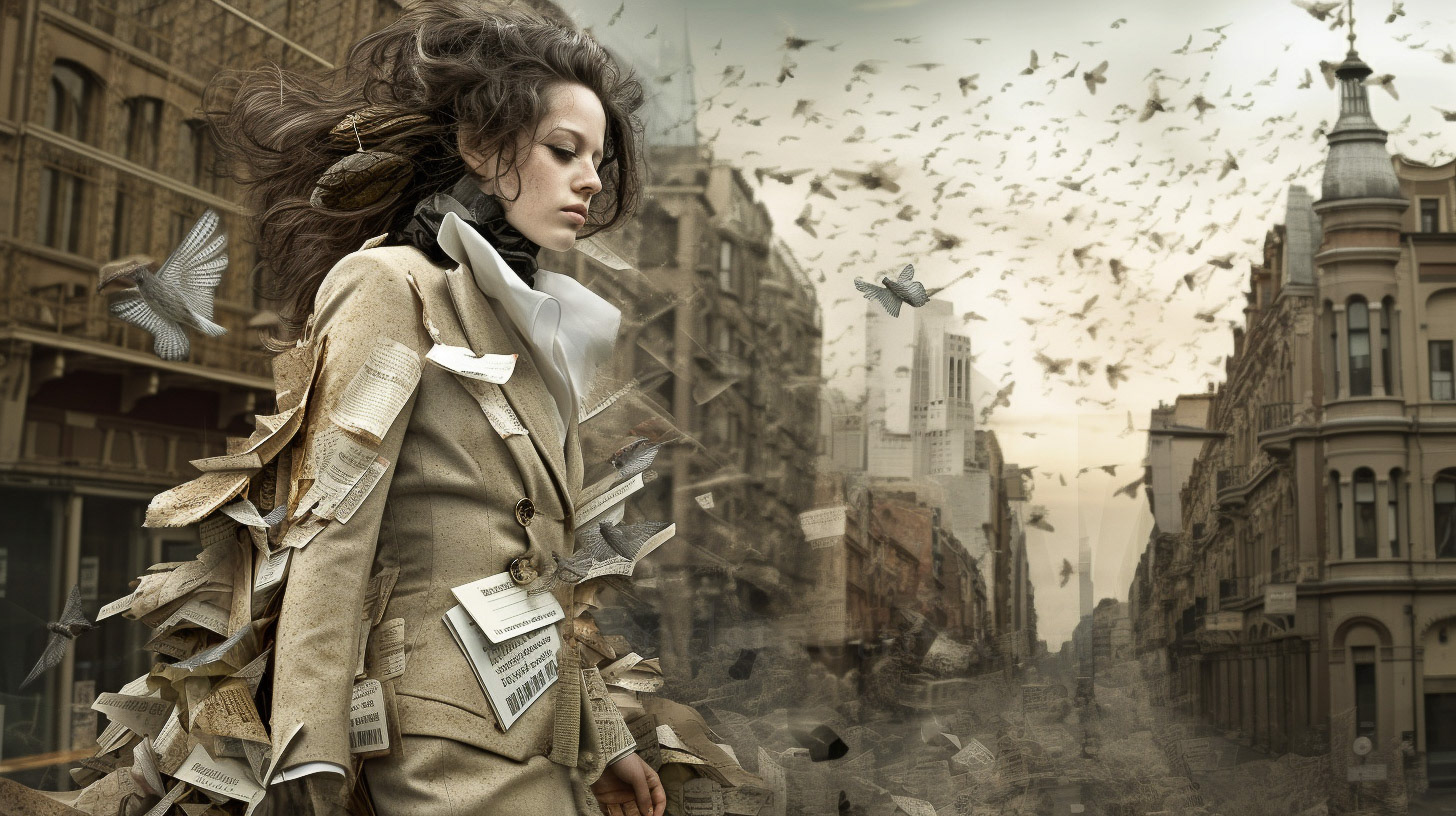
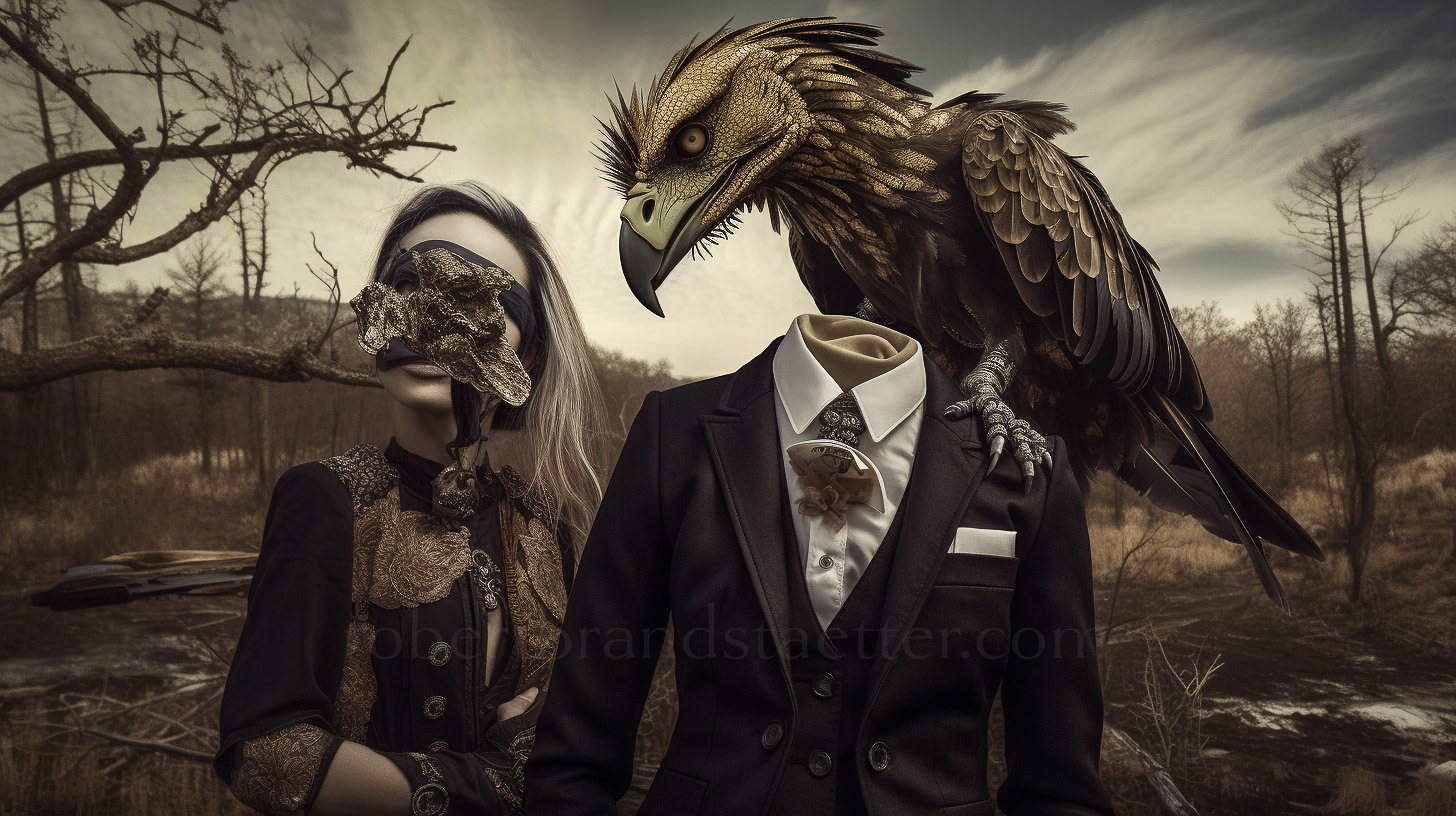
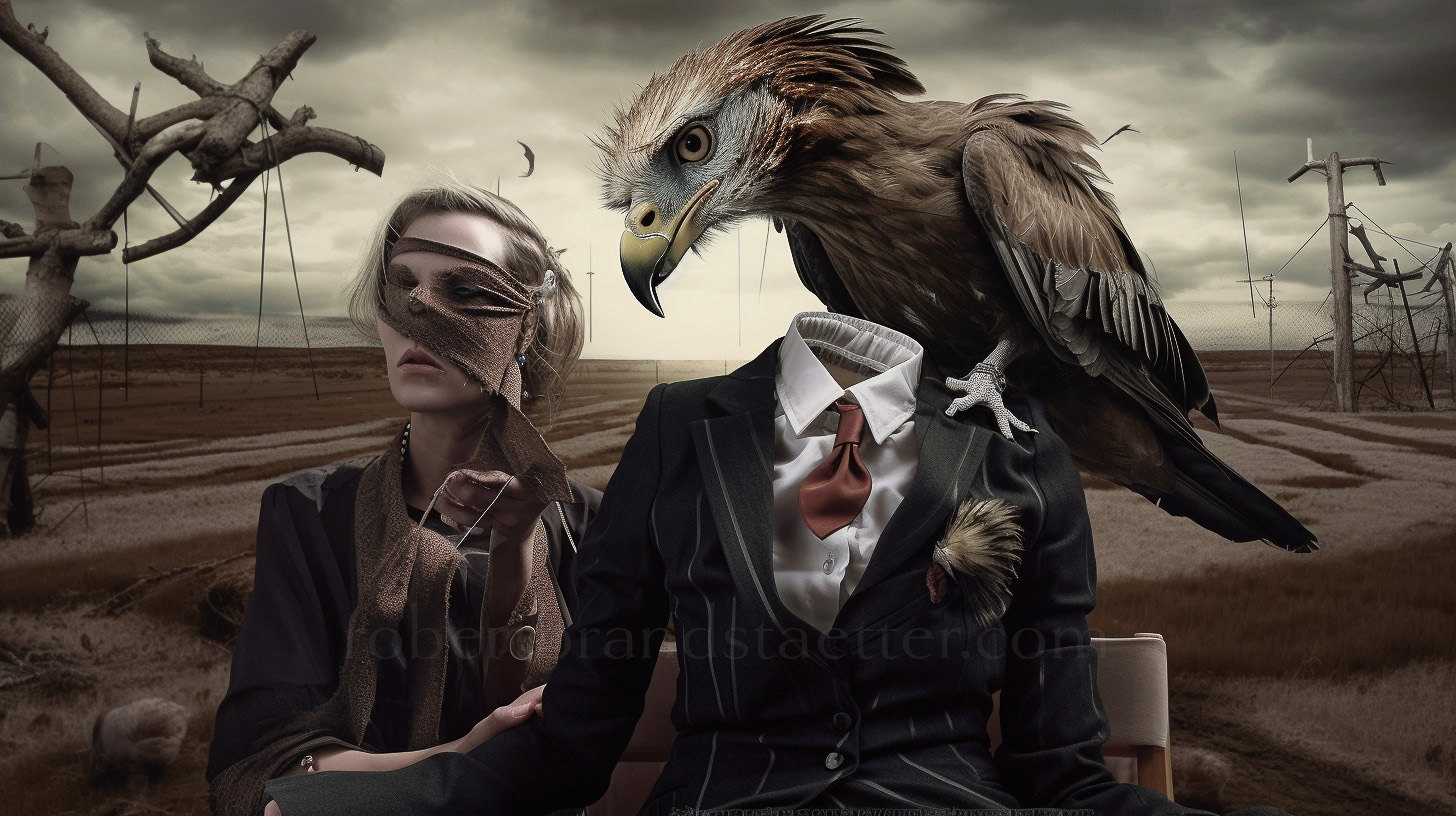
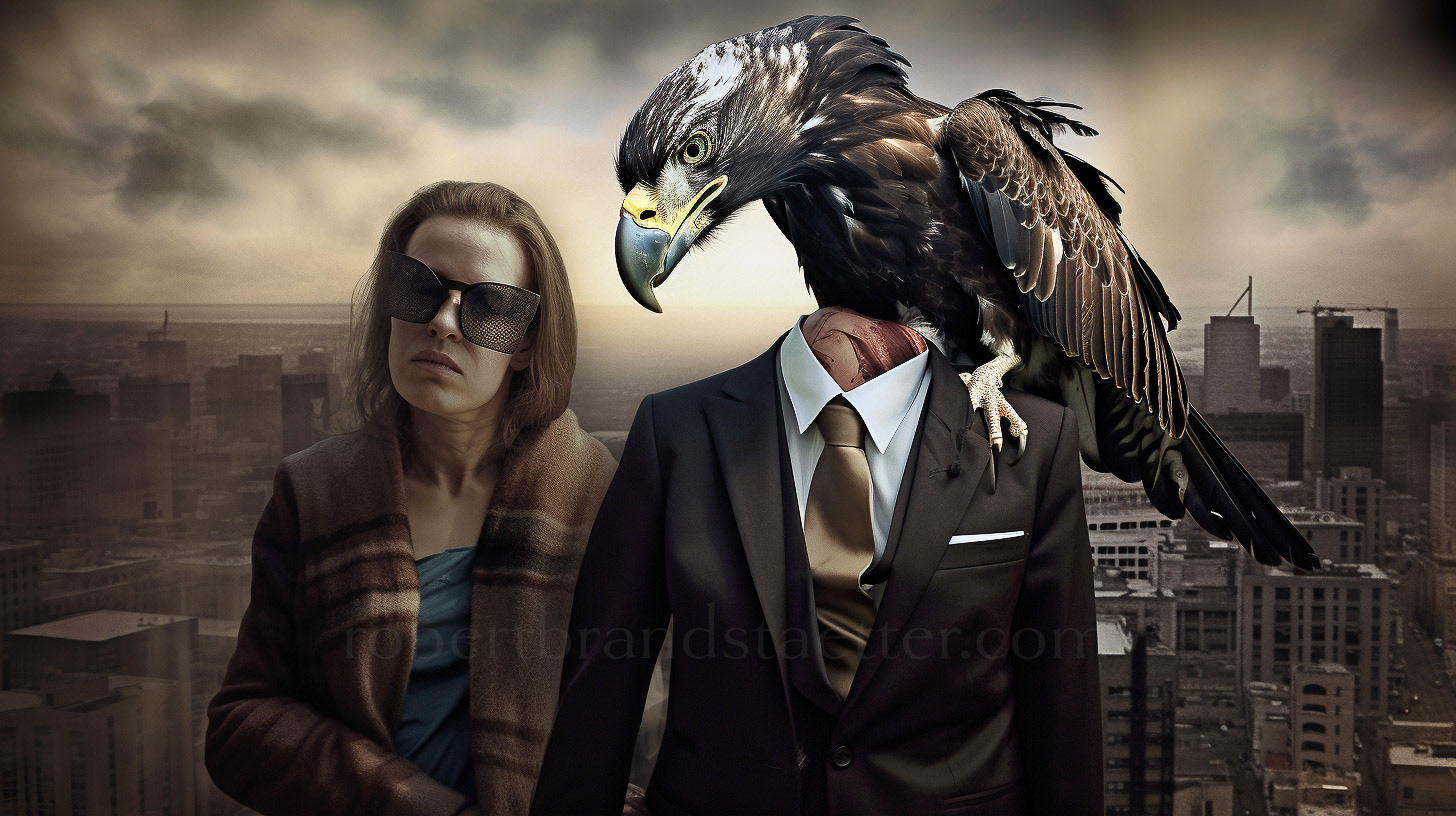
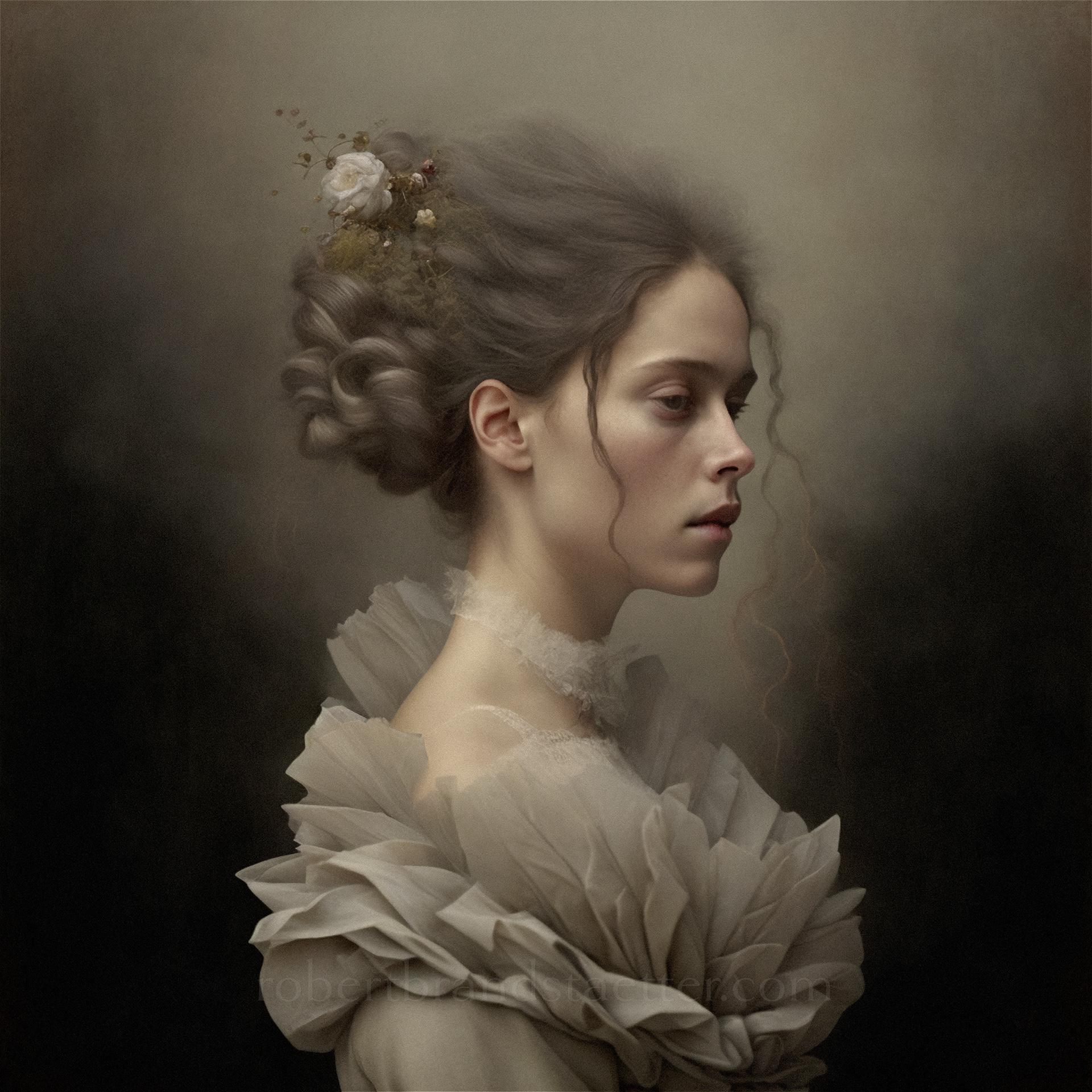
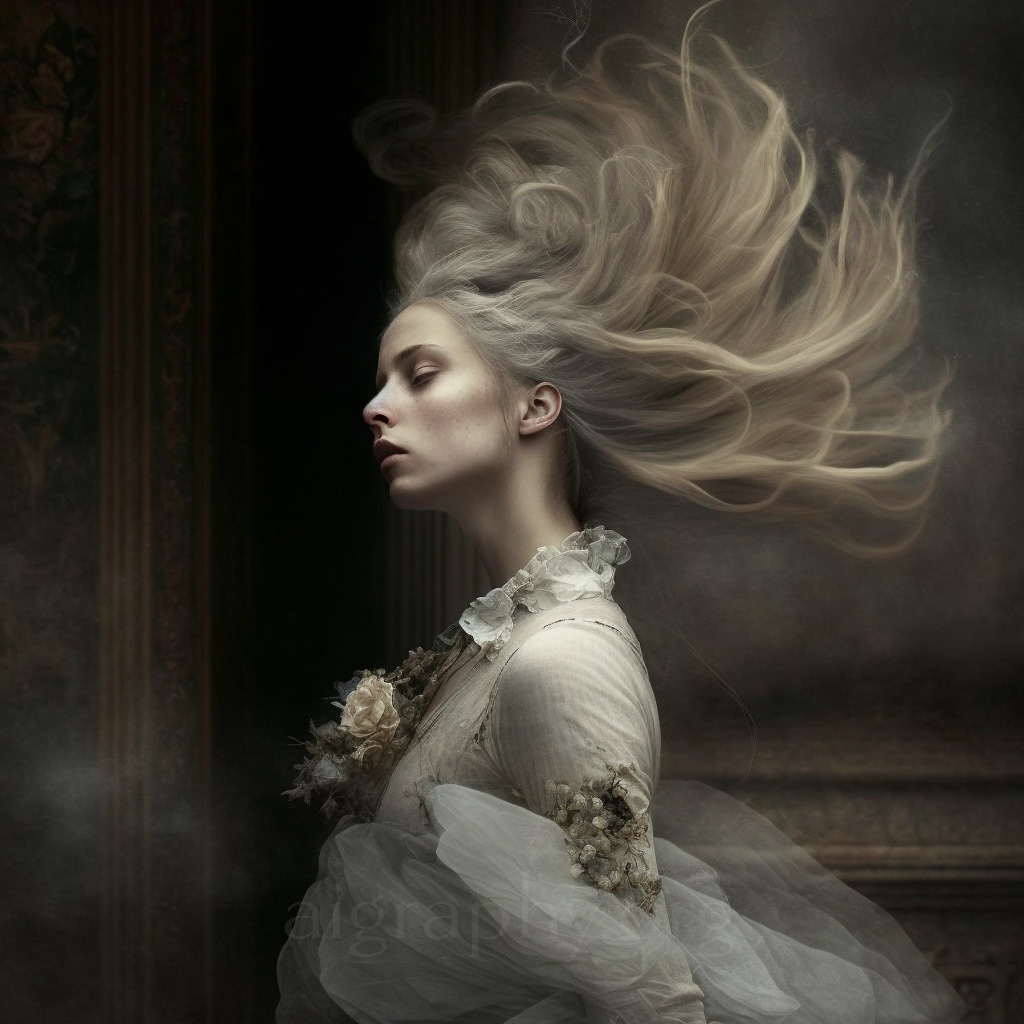
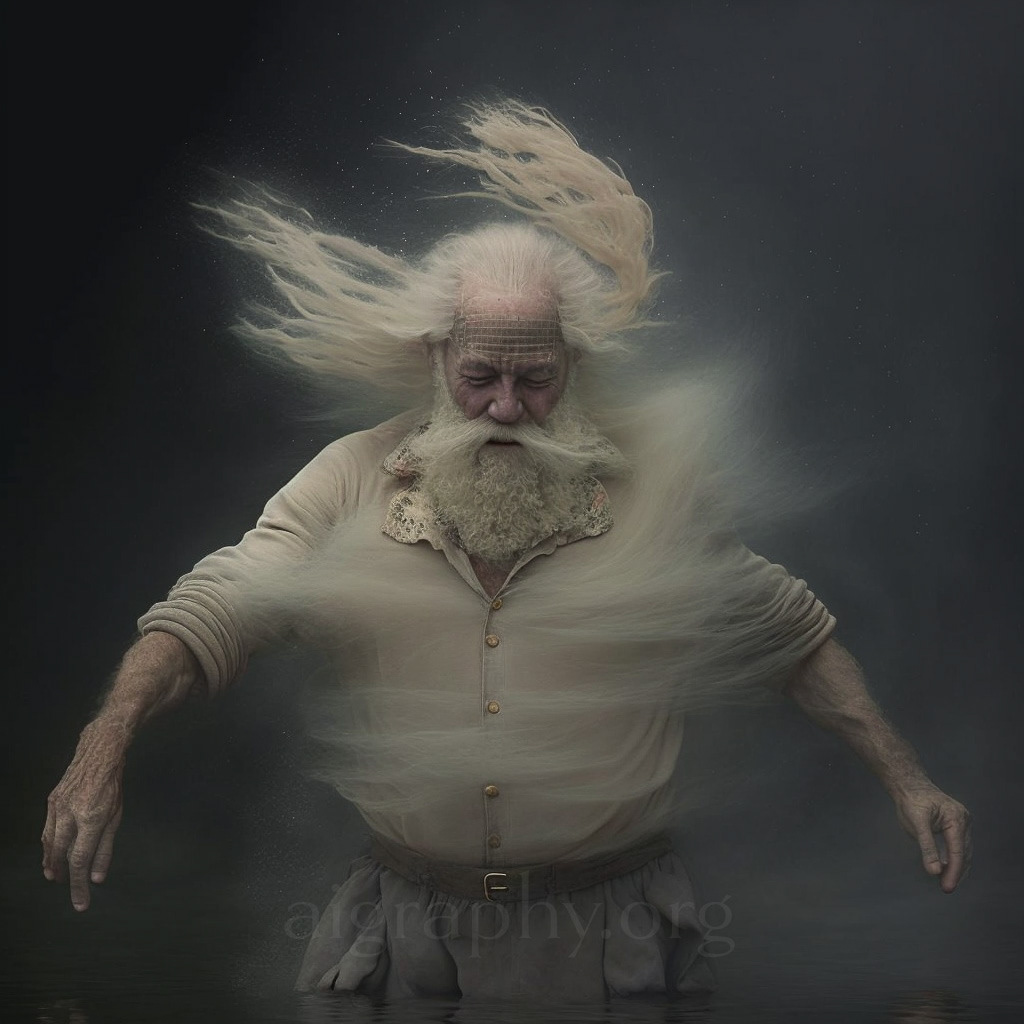
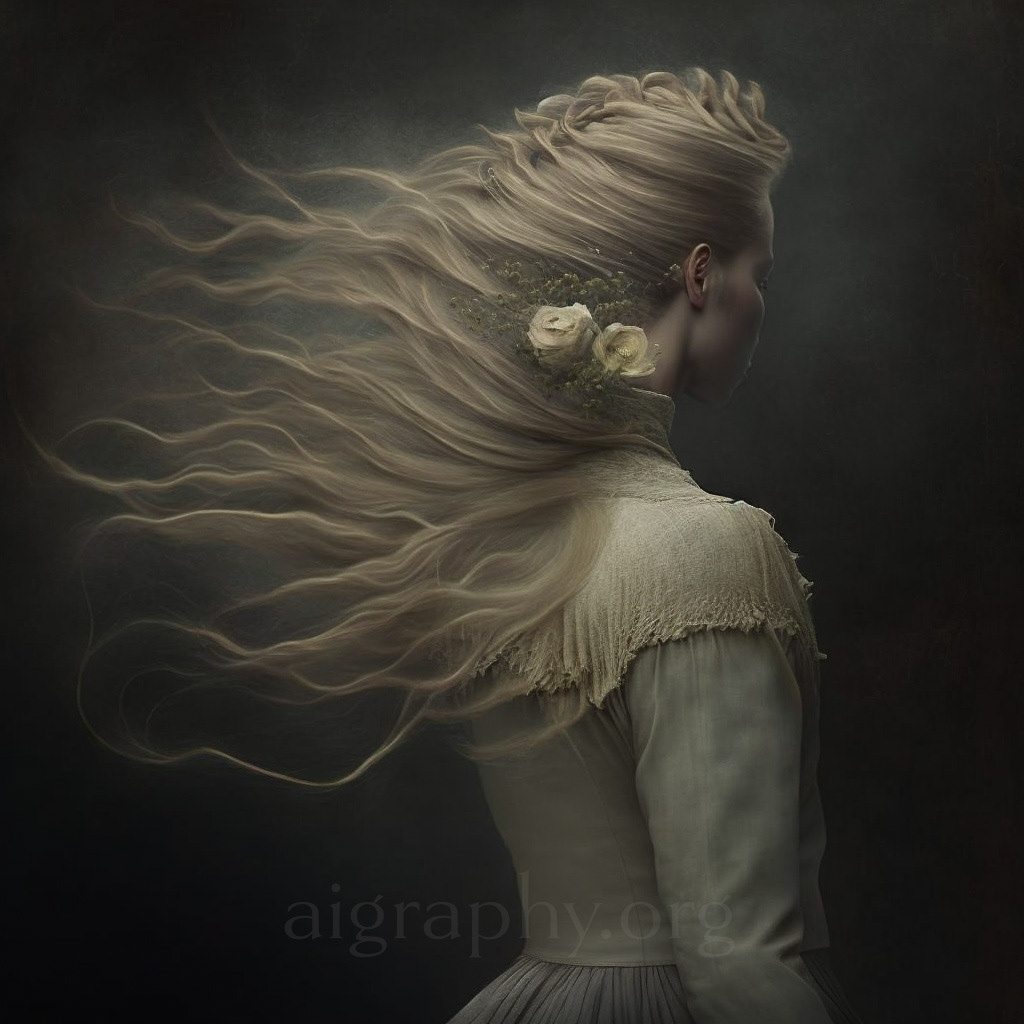
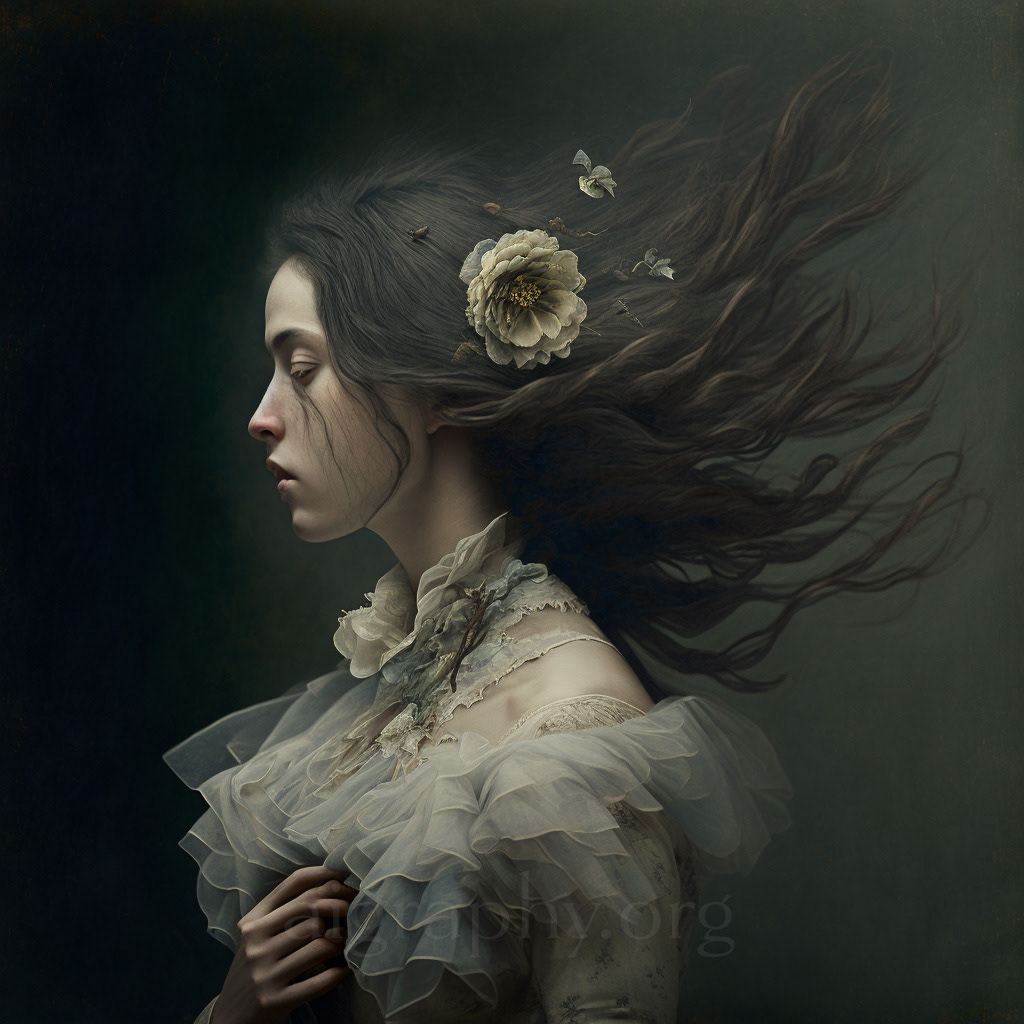
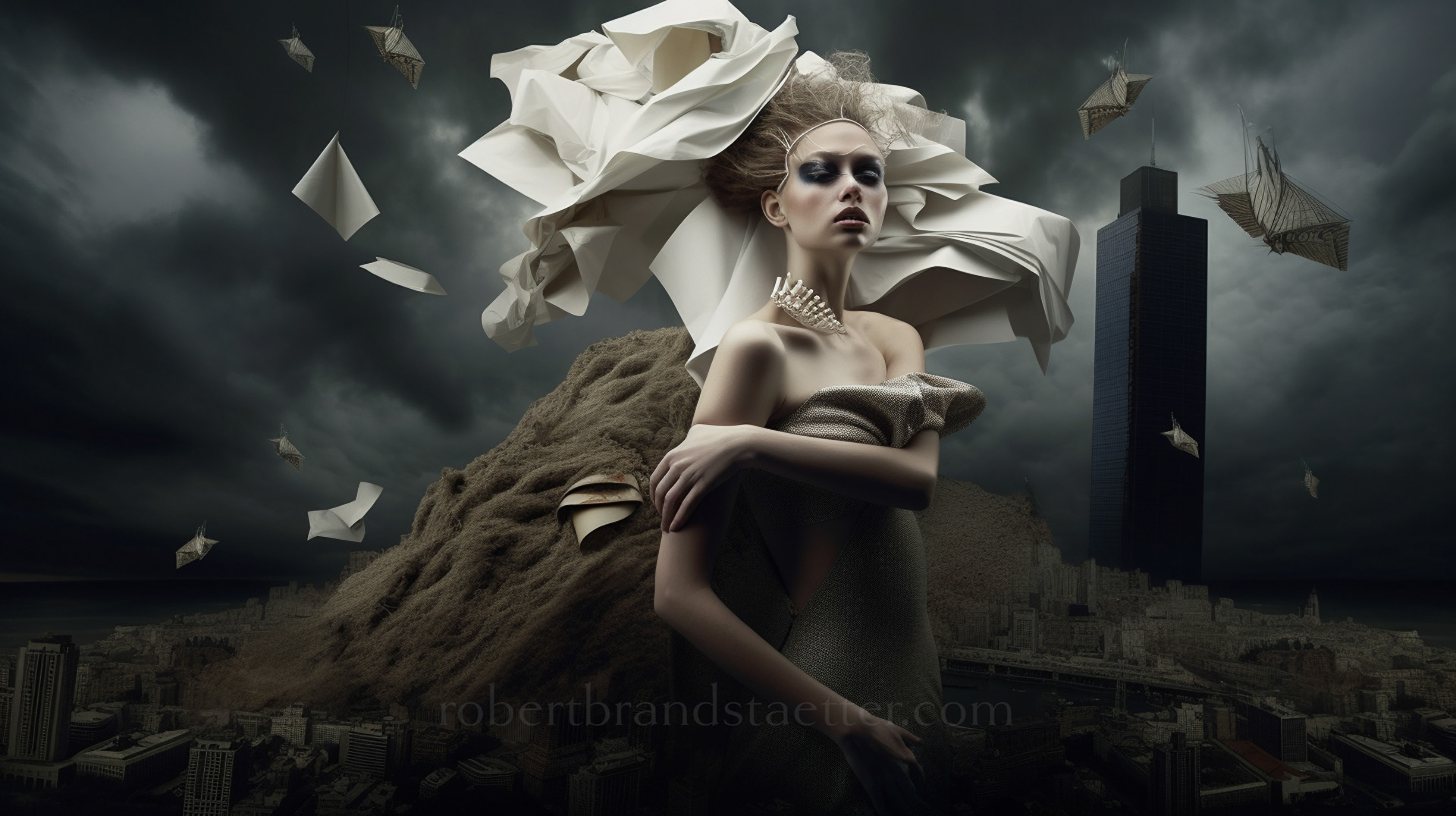
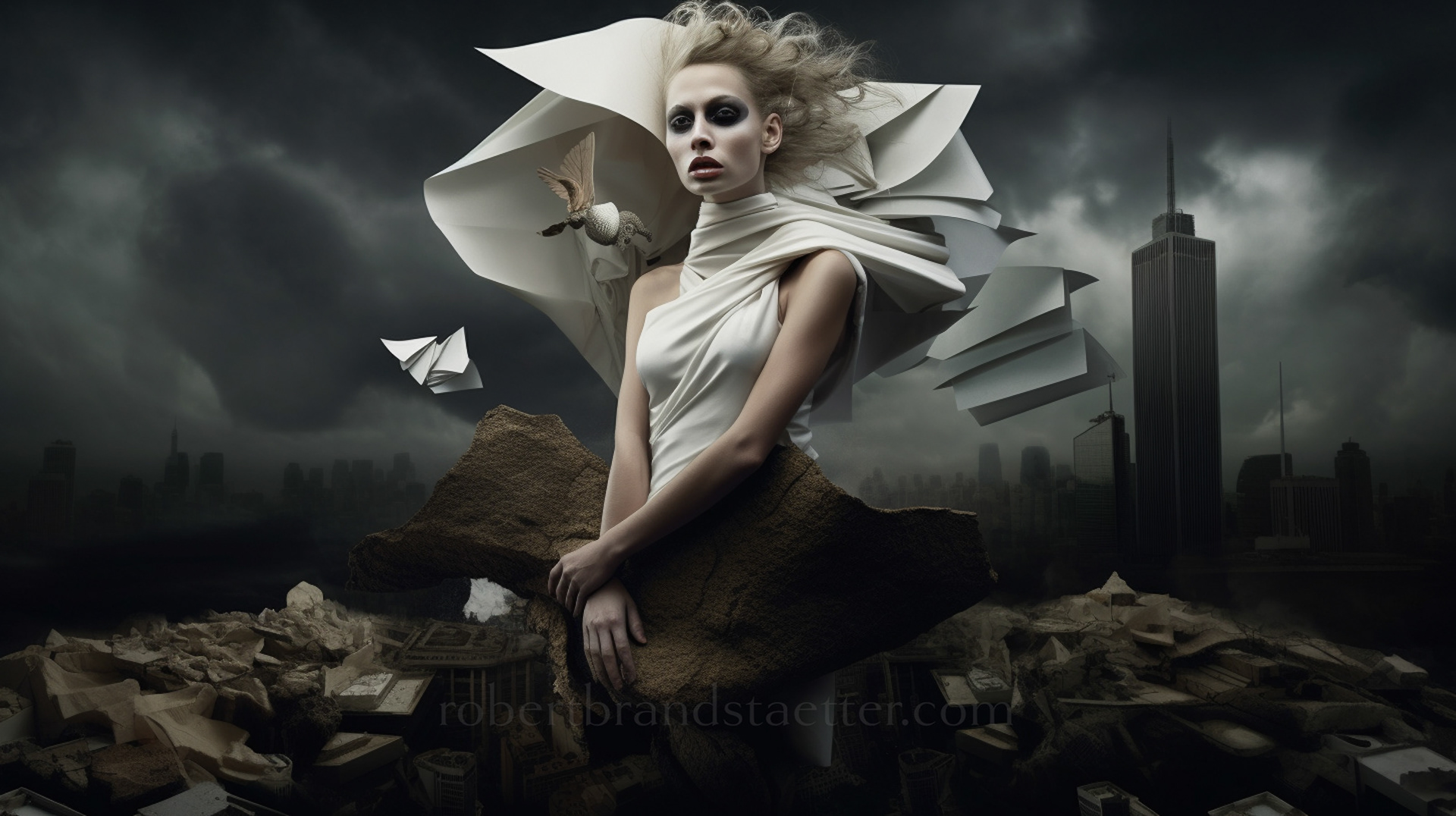
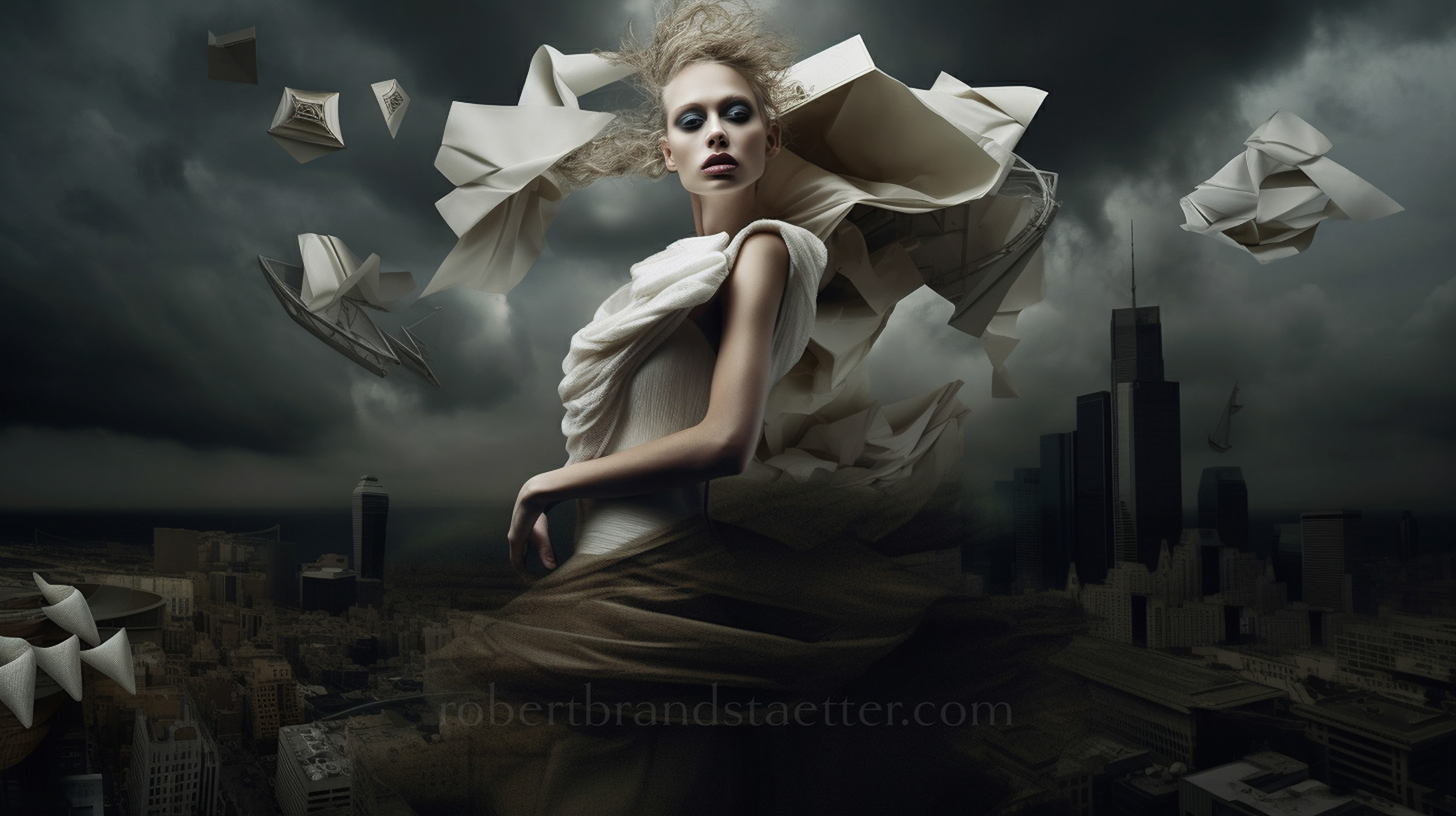
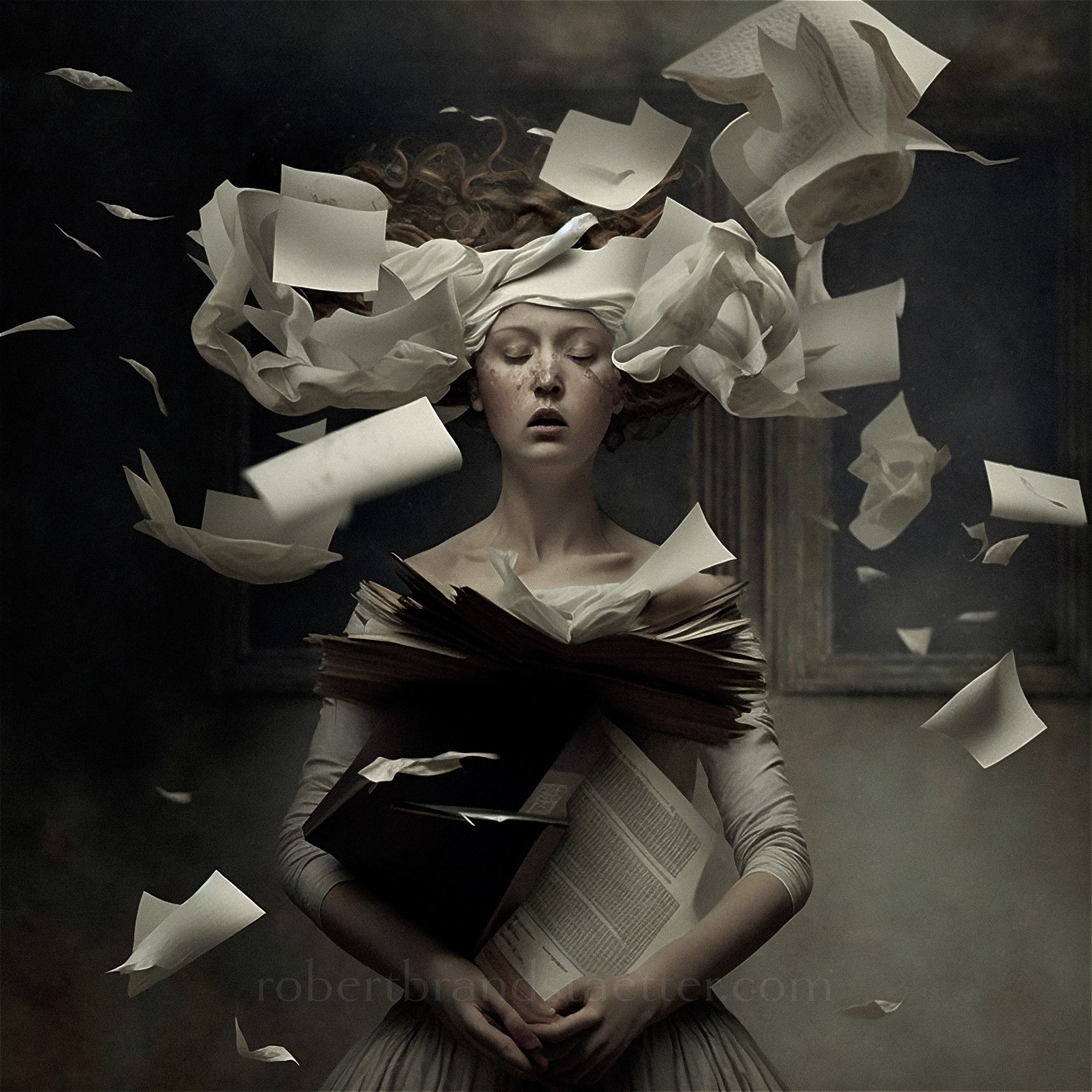
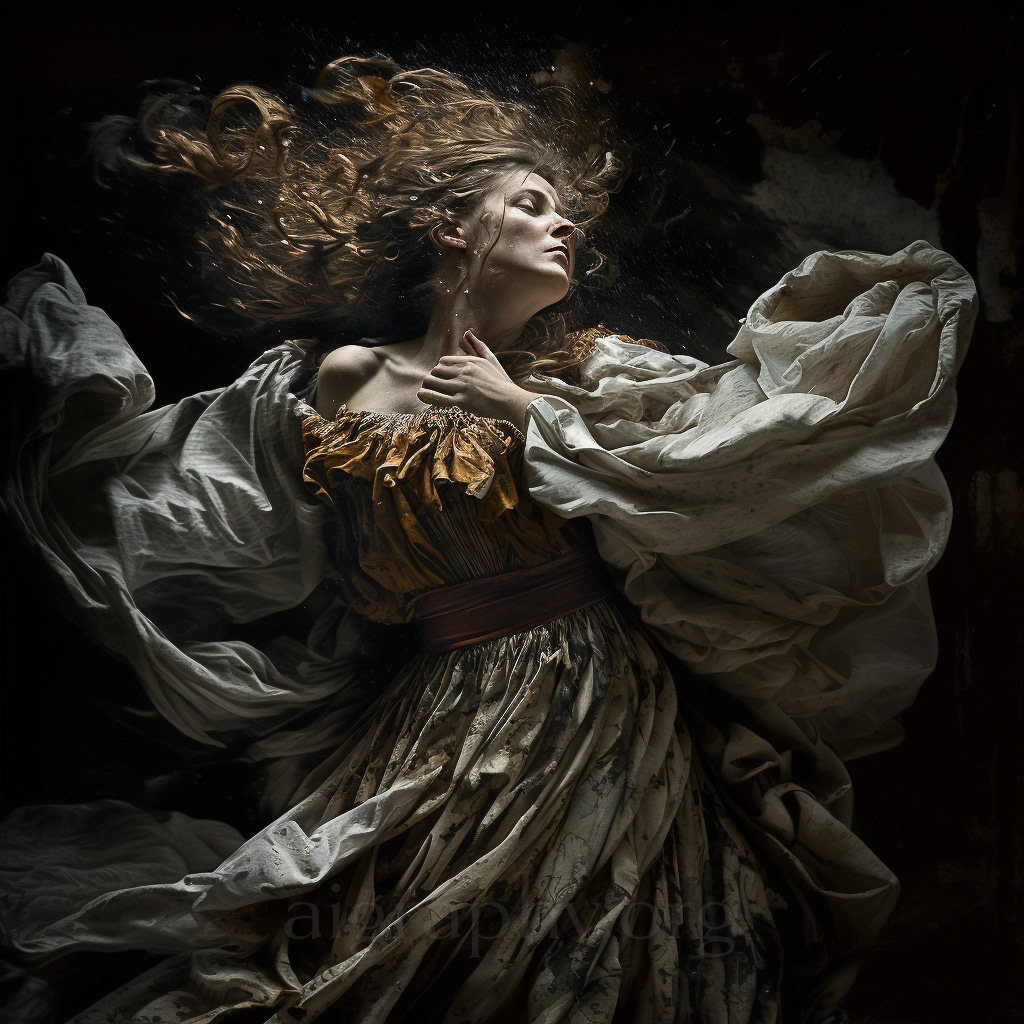
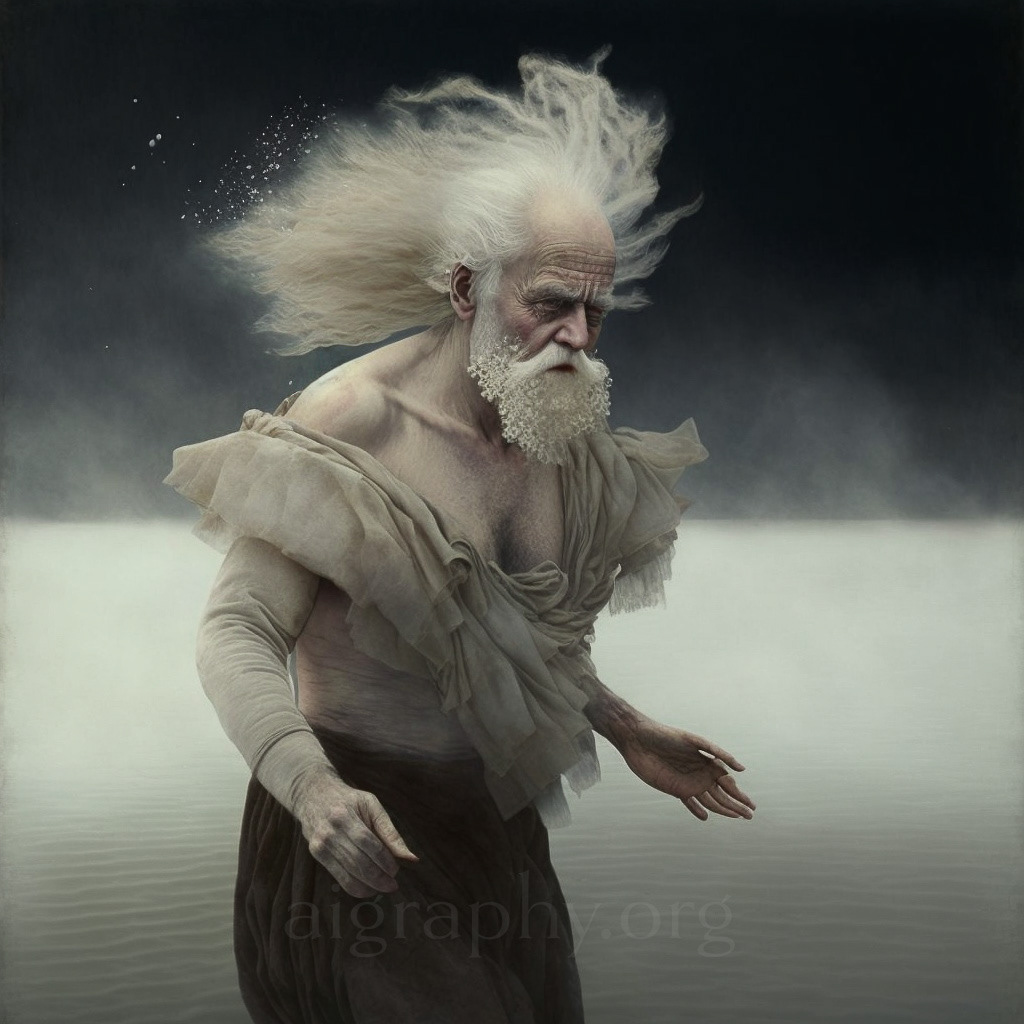
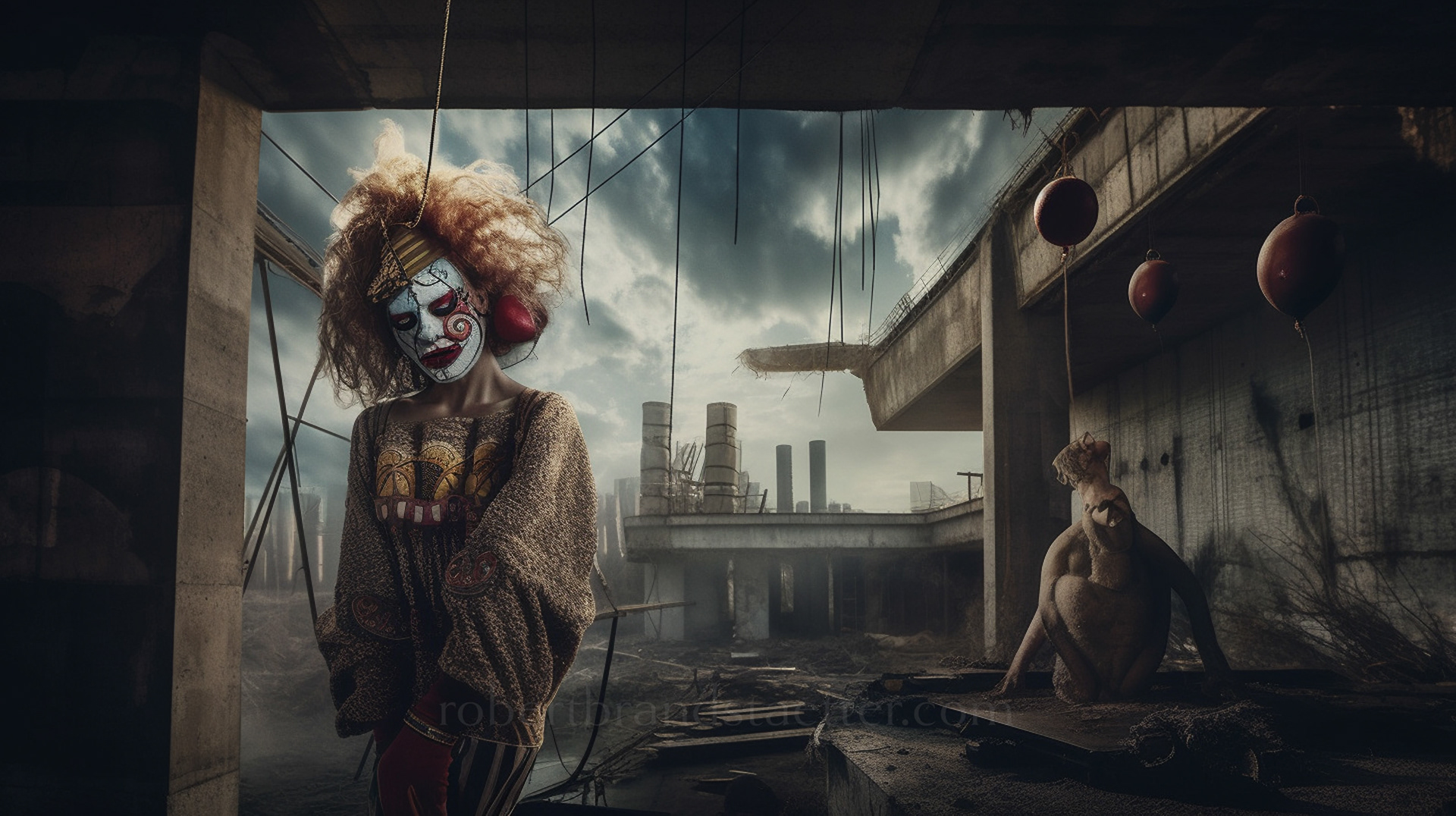
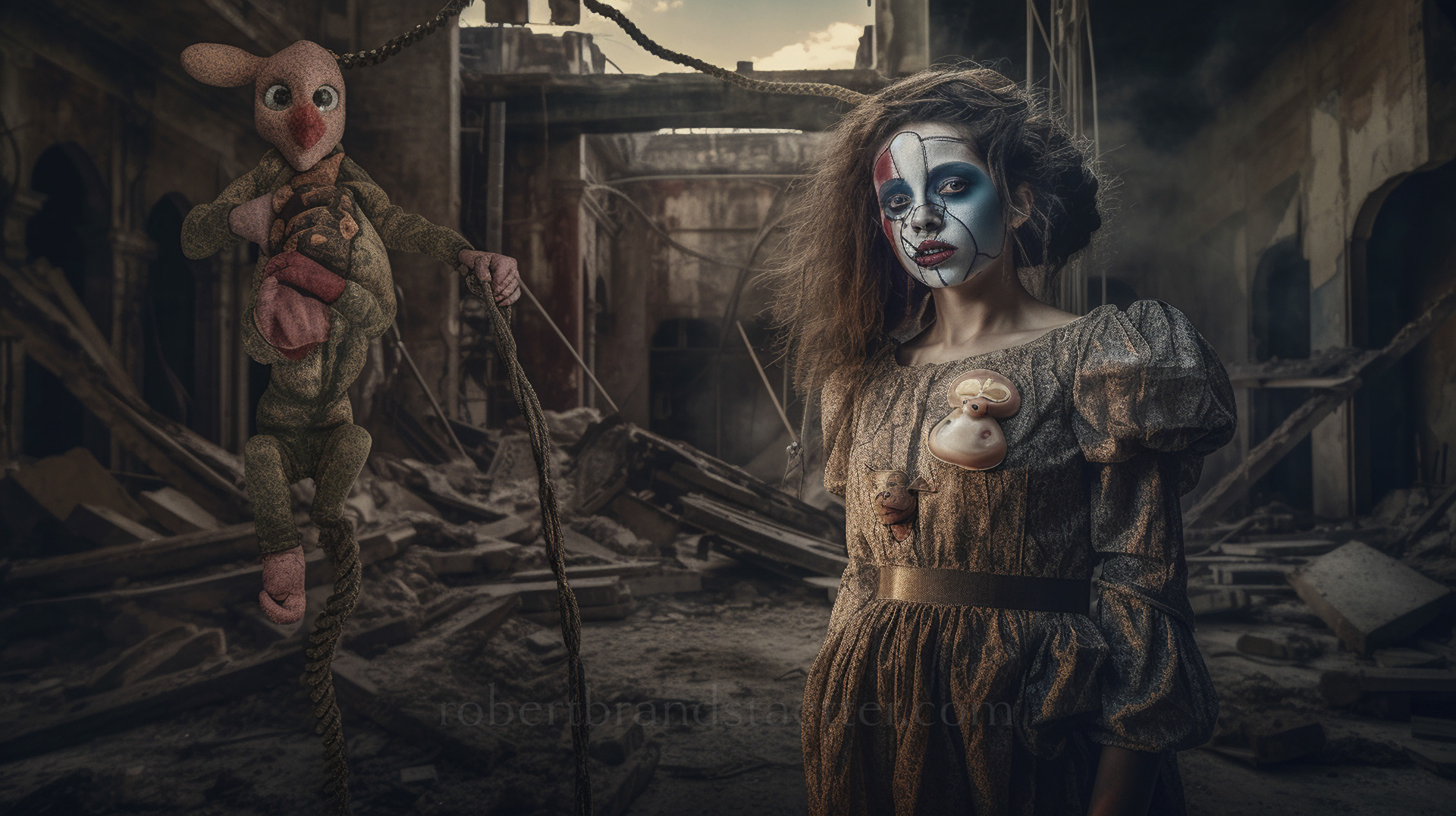
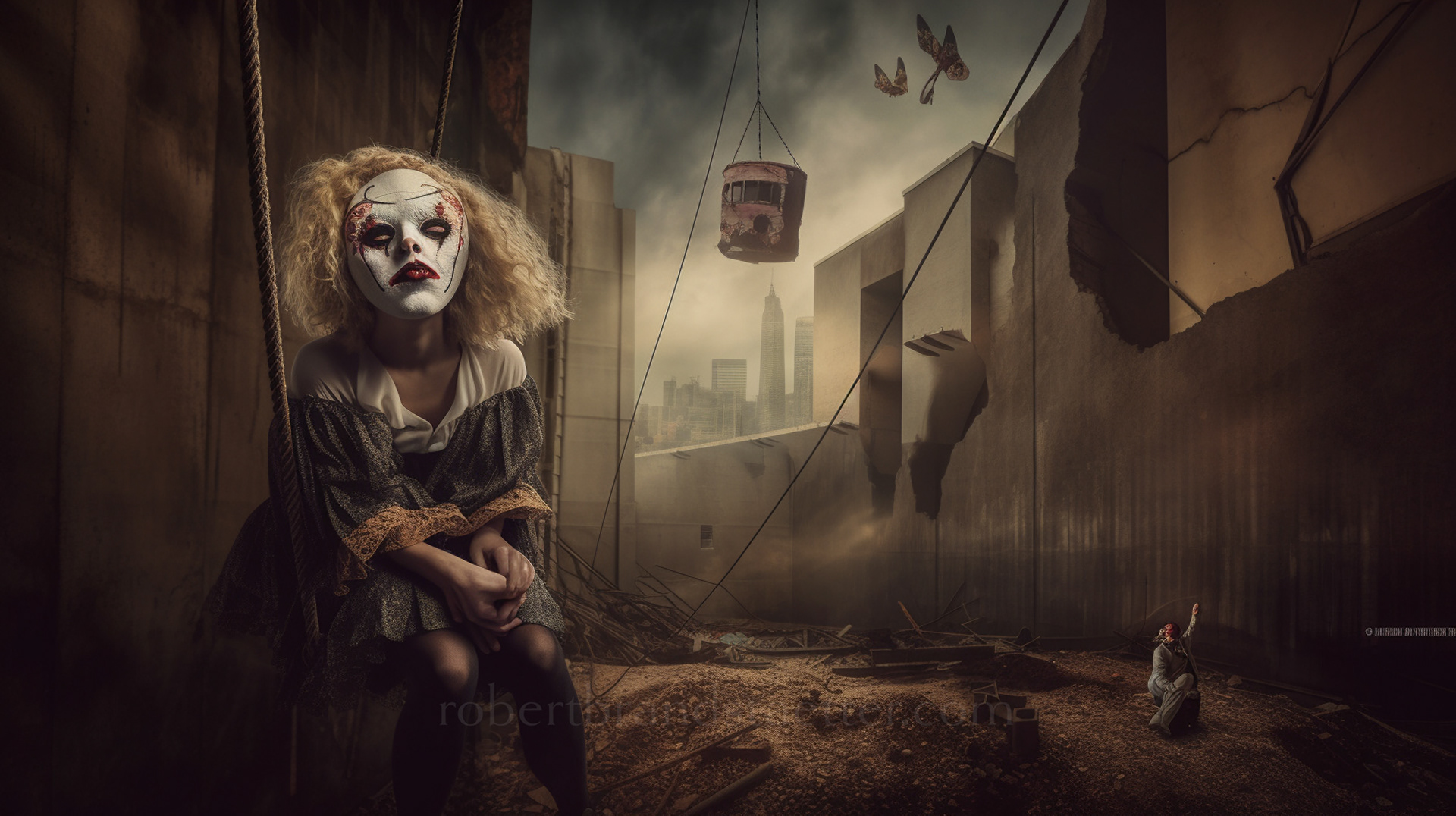
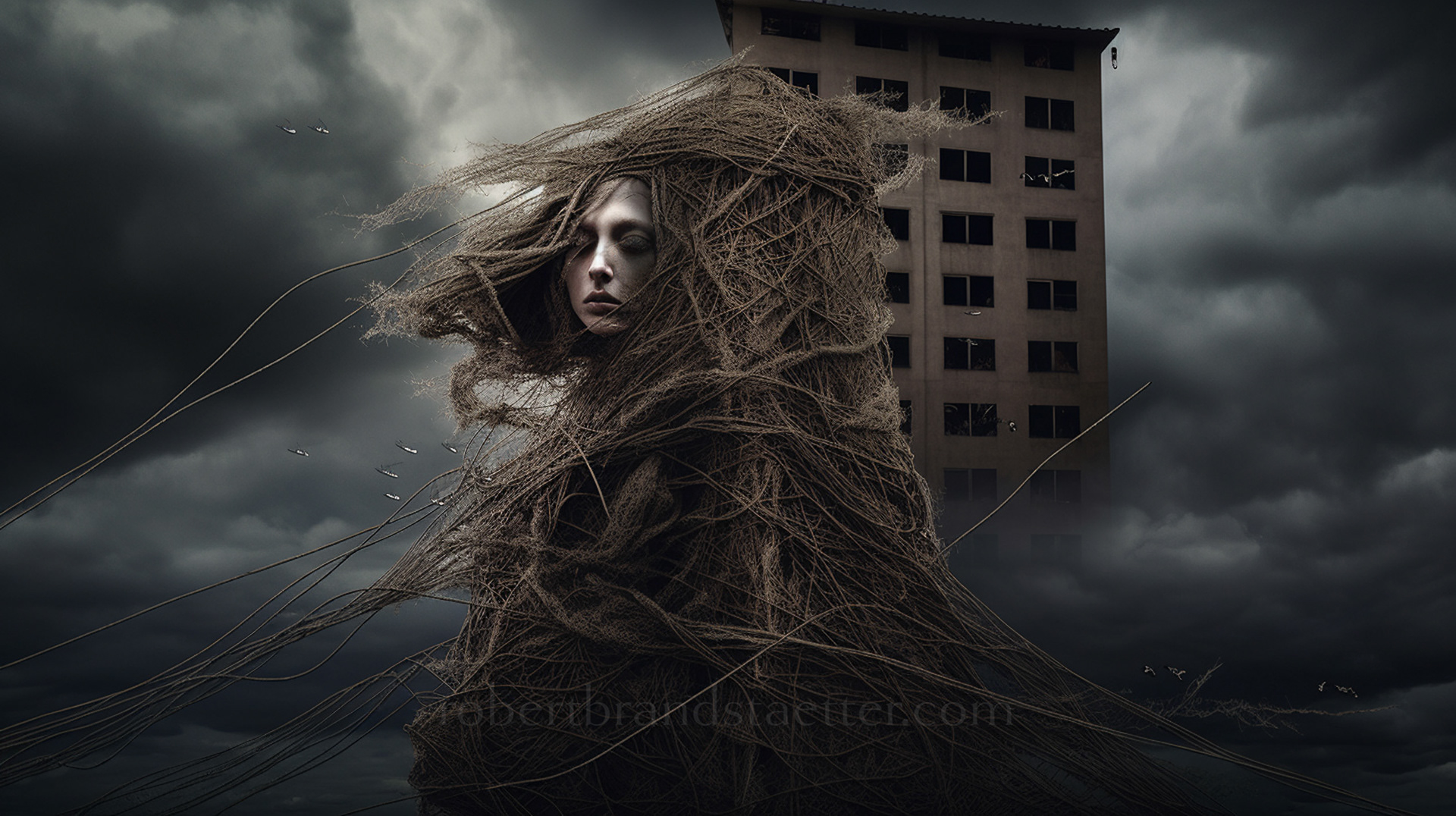
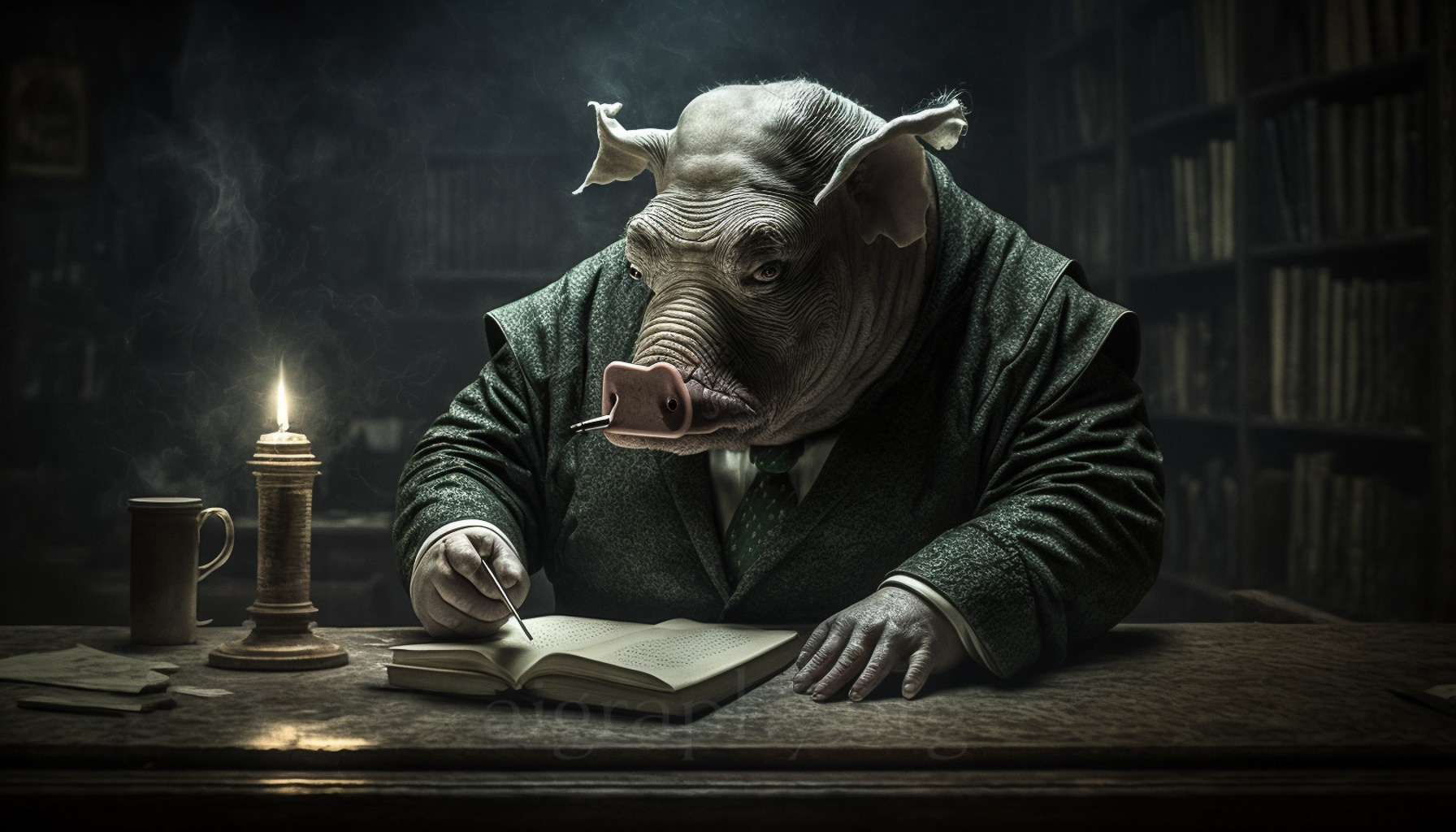
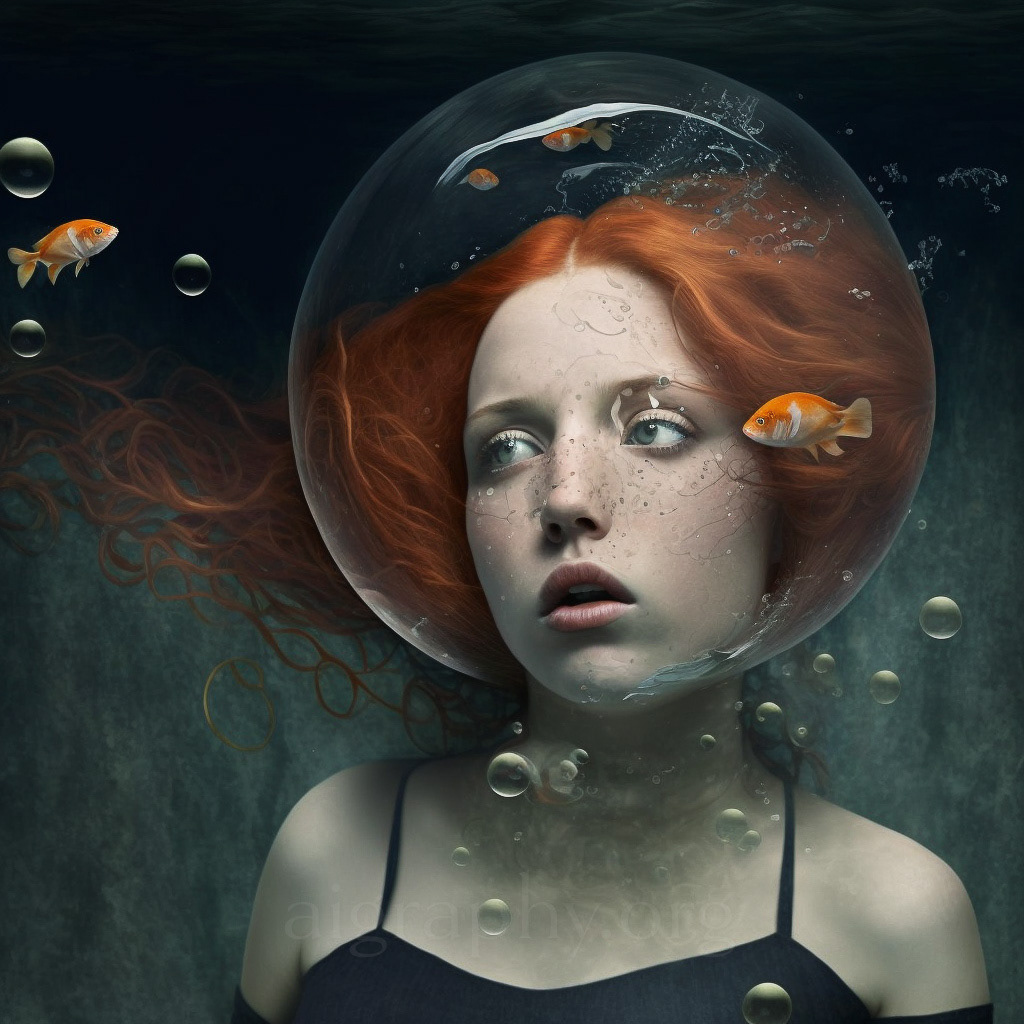
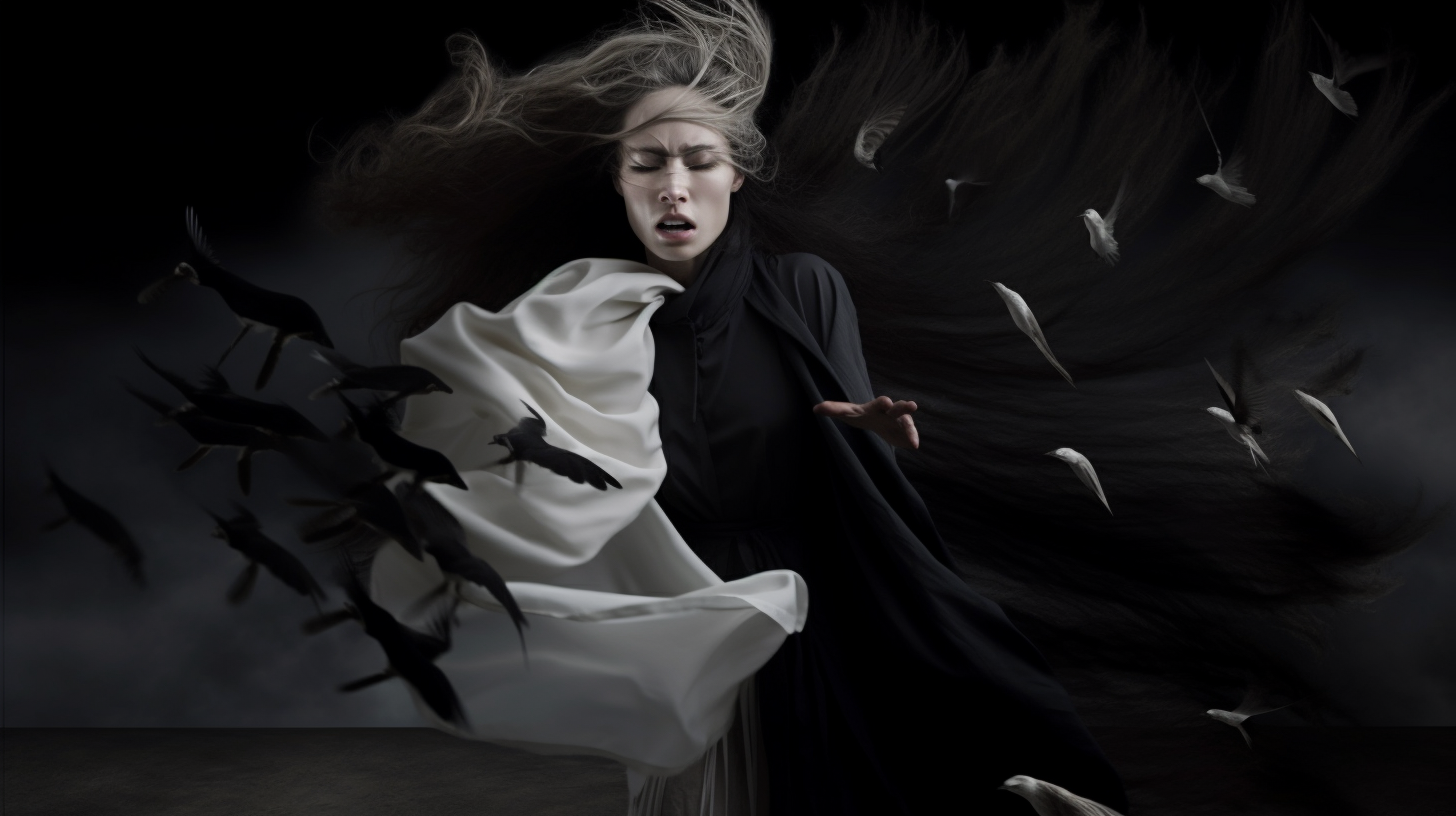
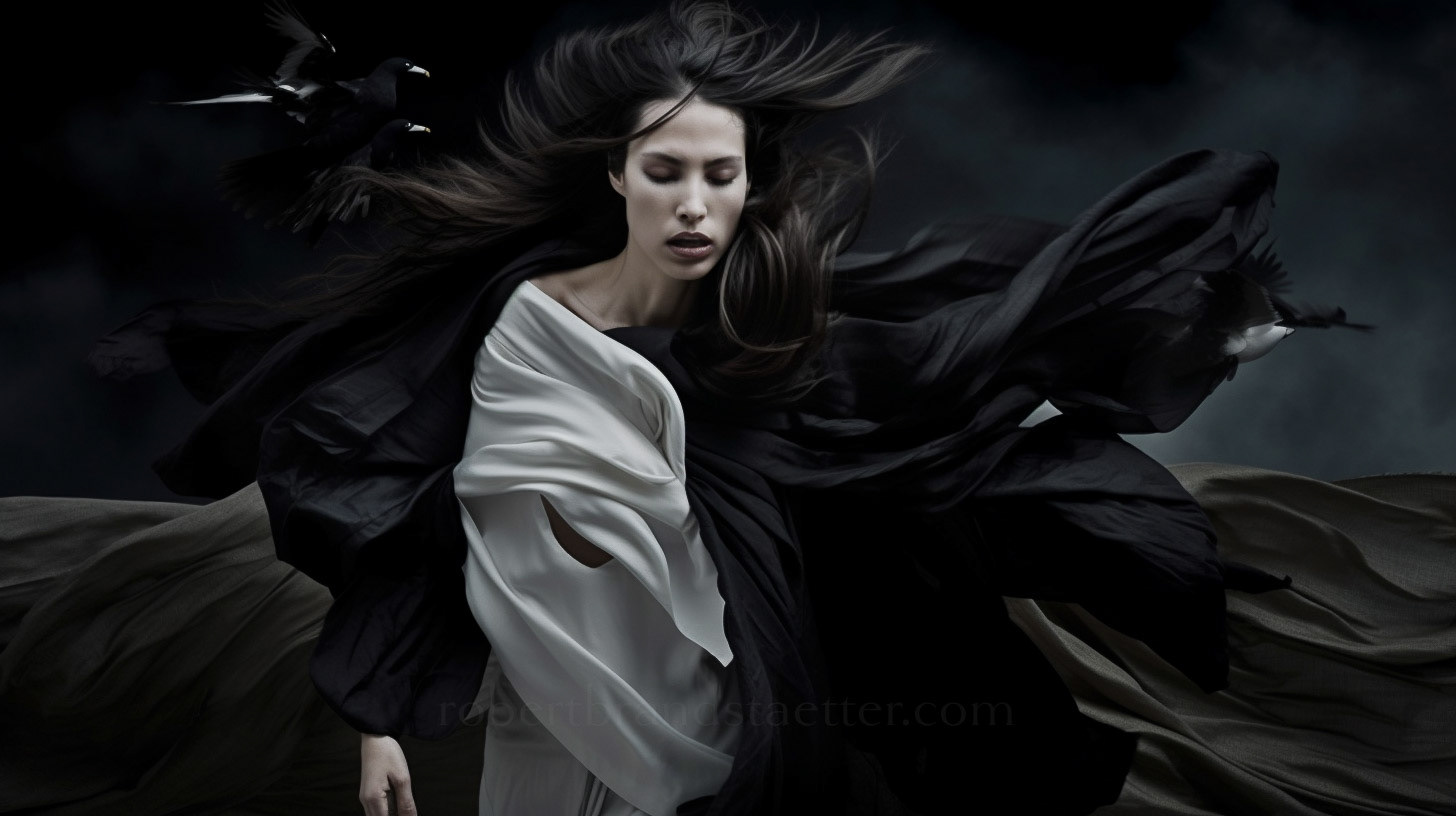
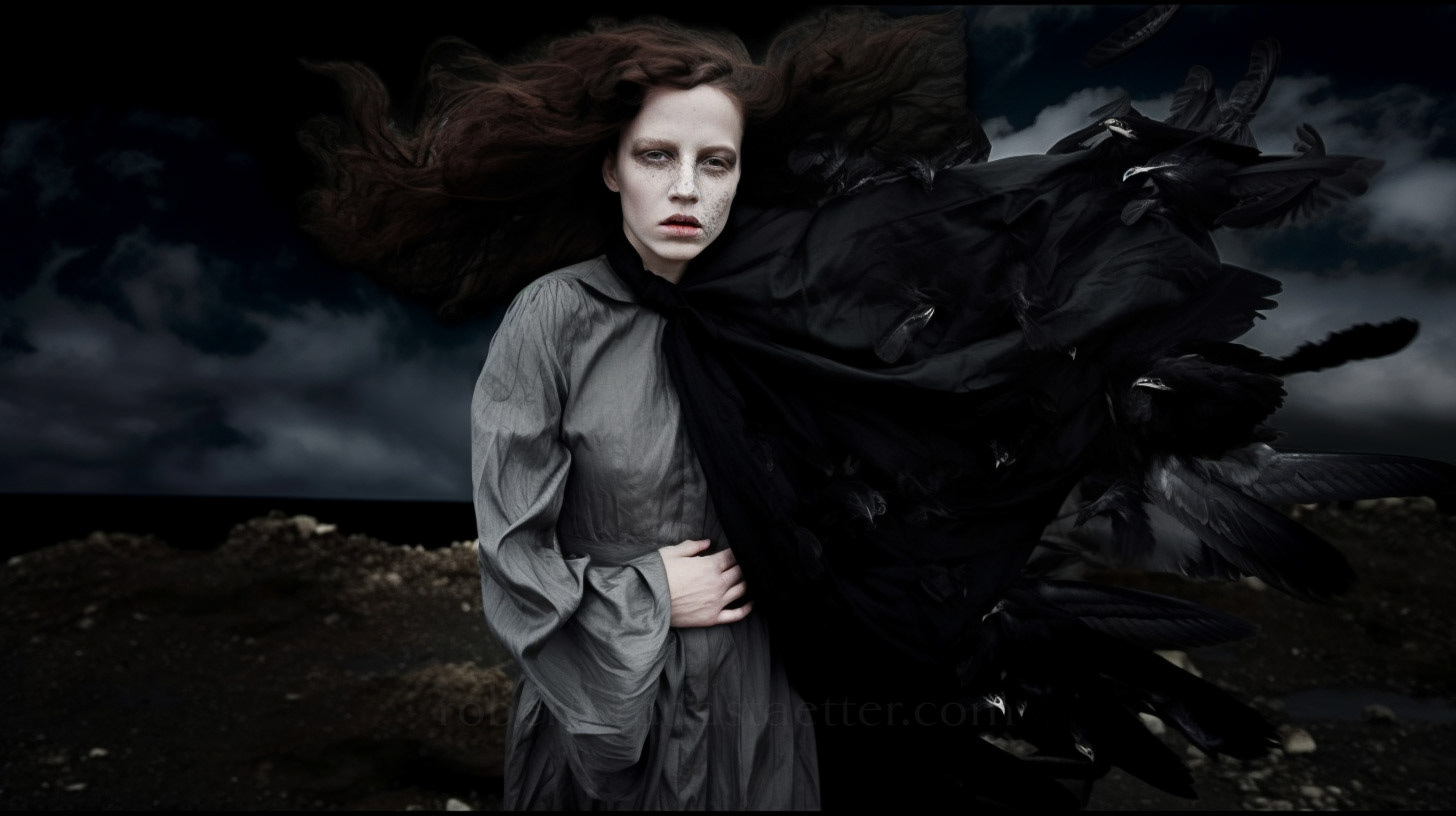
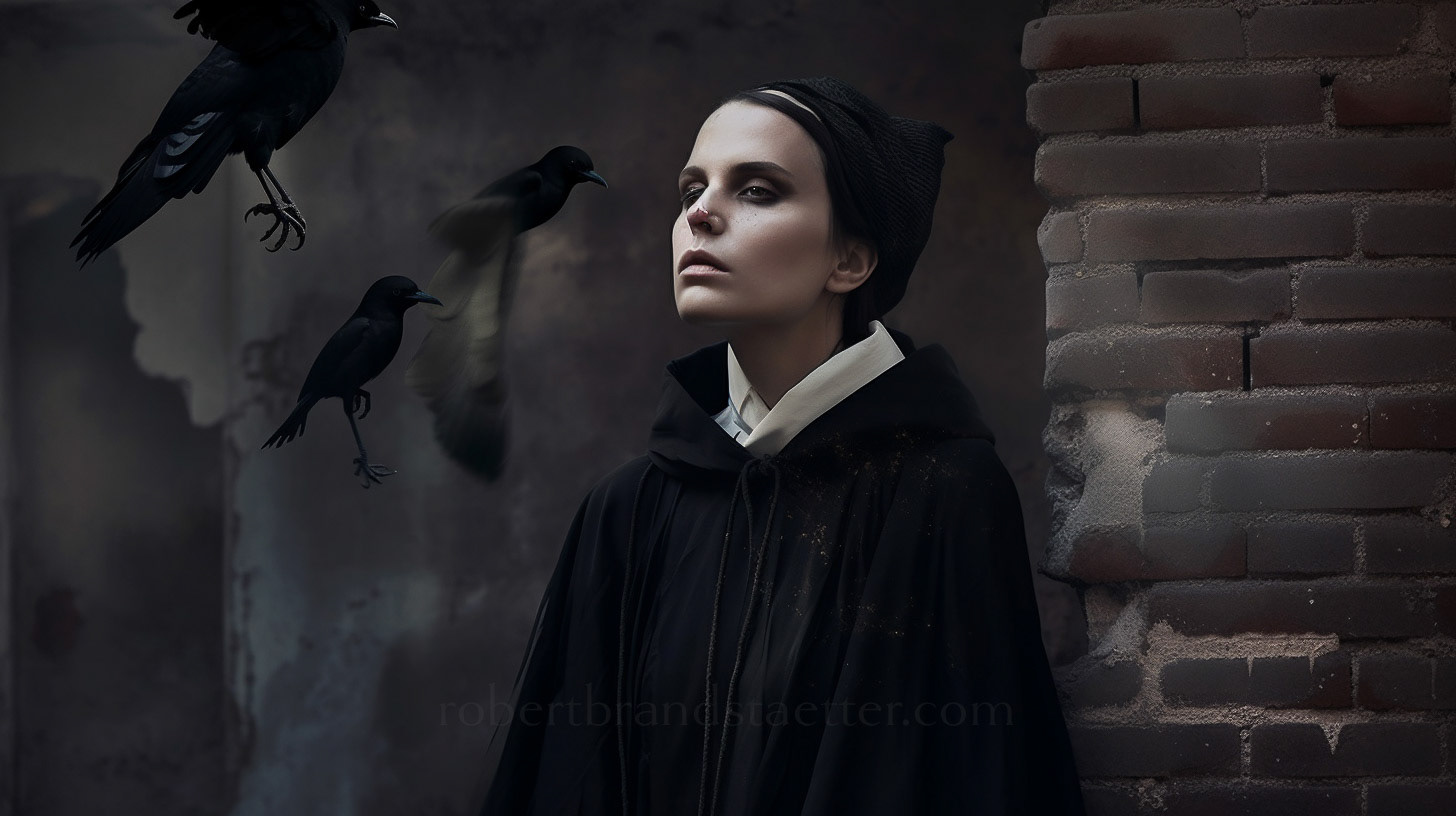
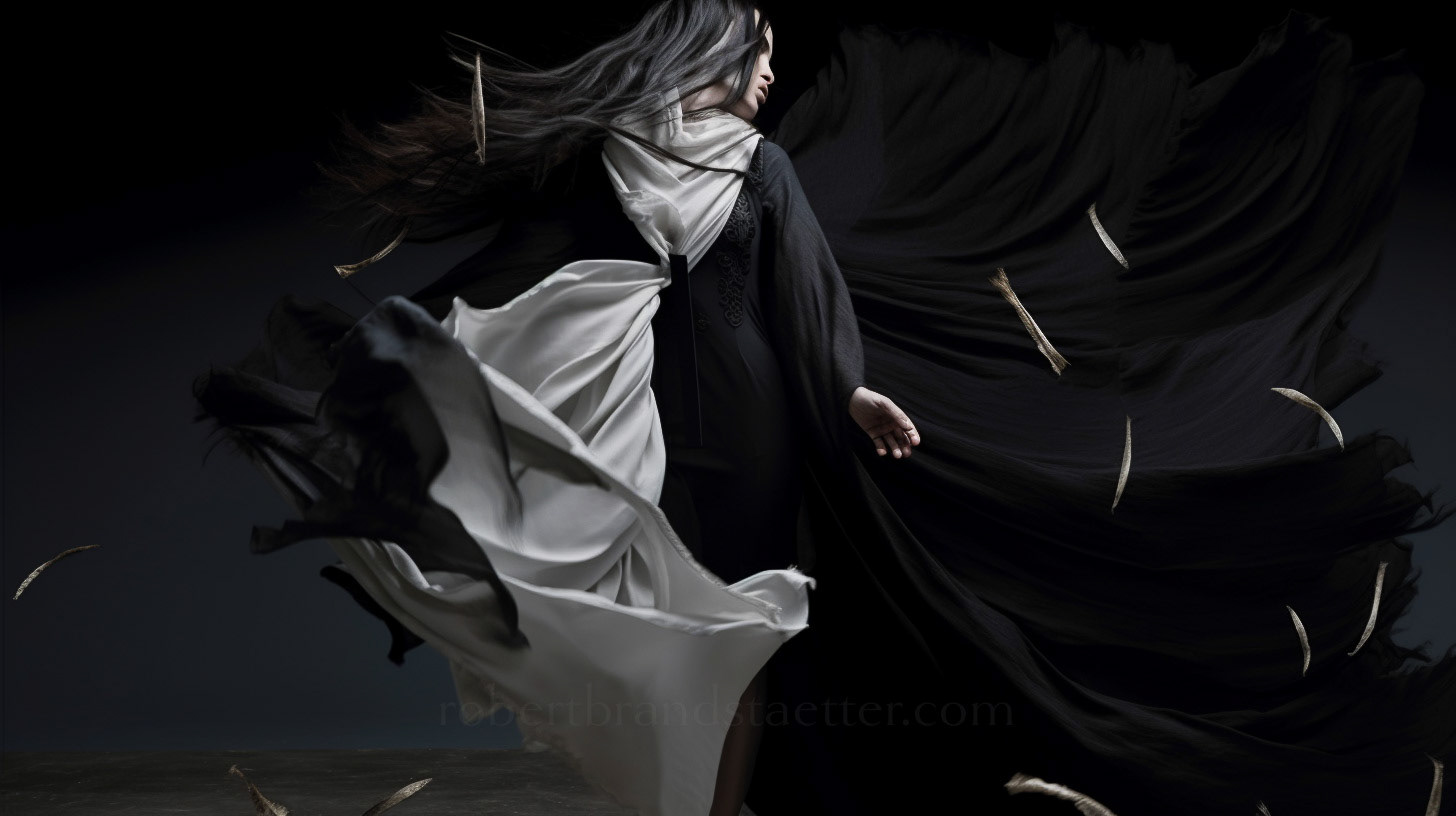
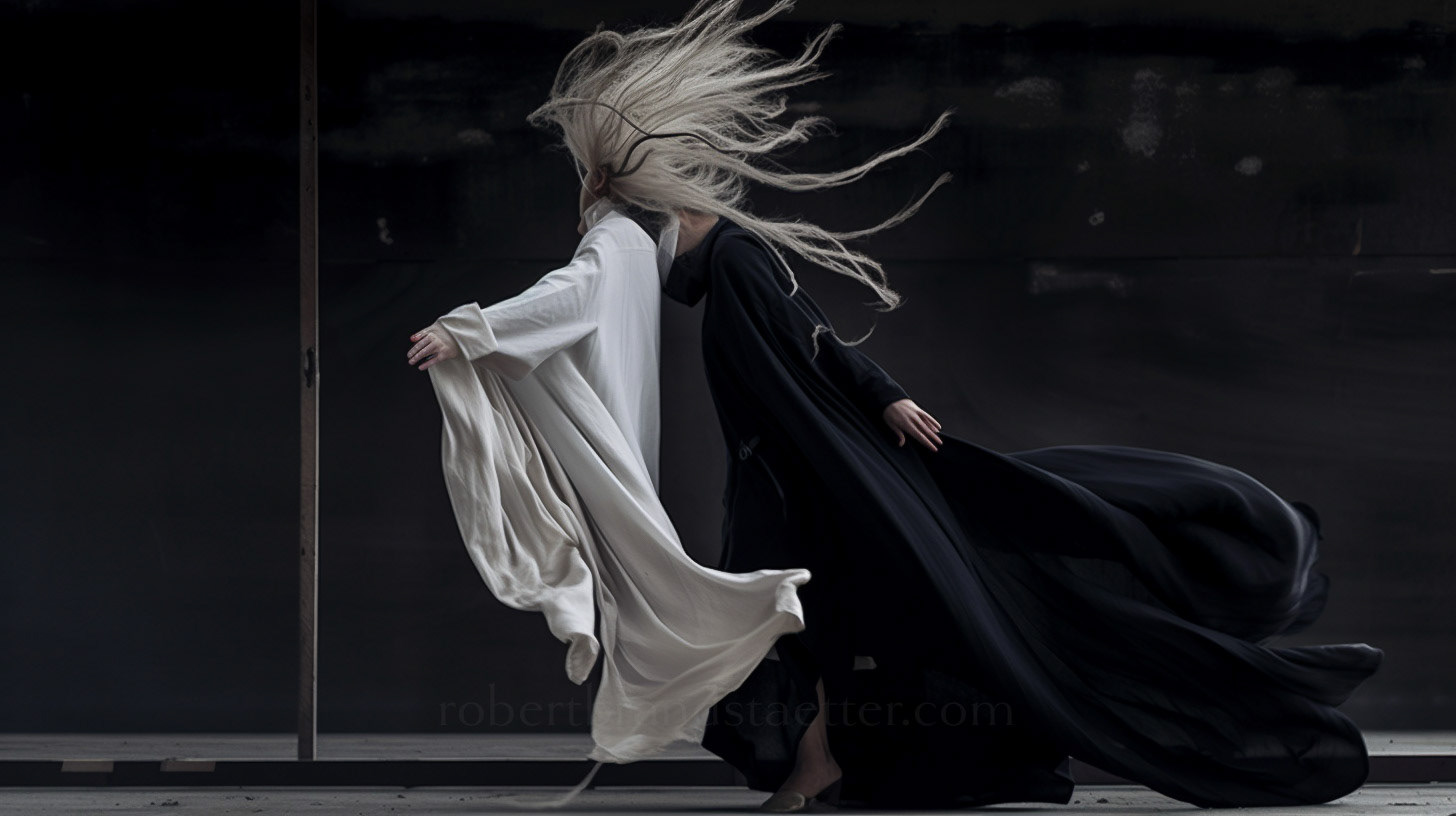
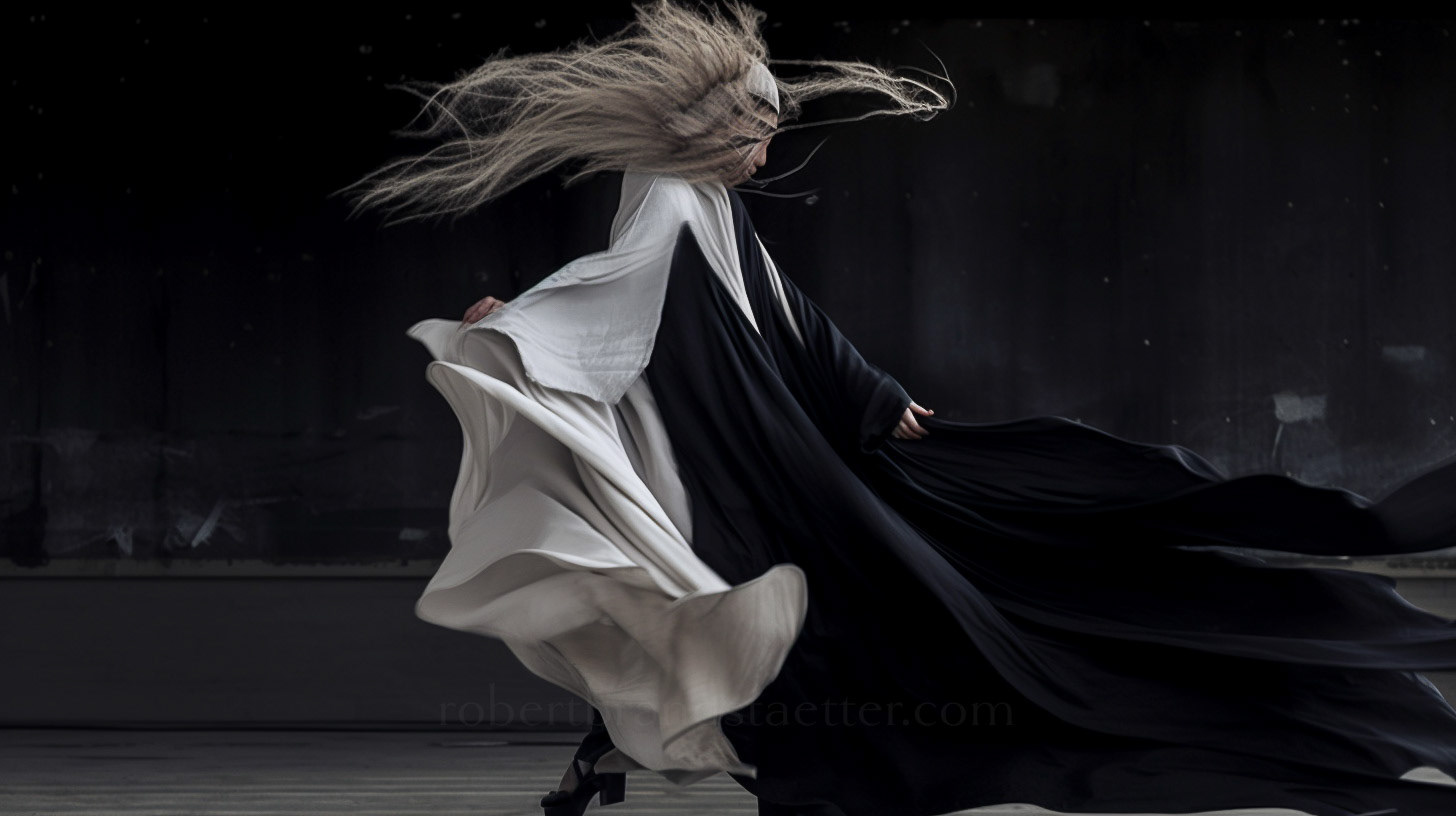

webmail
Thank you!

DISCLAIMER:
Information given at this site might neither be adequate nor correct.
Images are based on noise diffusion AI models.
Robert M. Brandstaetter | Austria | Vienna 1090 | Guenthergasse 3
studioatrobertbrandstaetterdotcom
instagram
